What Is Data Cleaning?
Data cleaning is the process of cleansing, scrubbing, and preparing data for storage and analysis. Learn why that’s important and more.
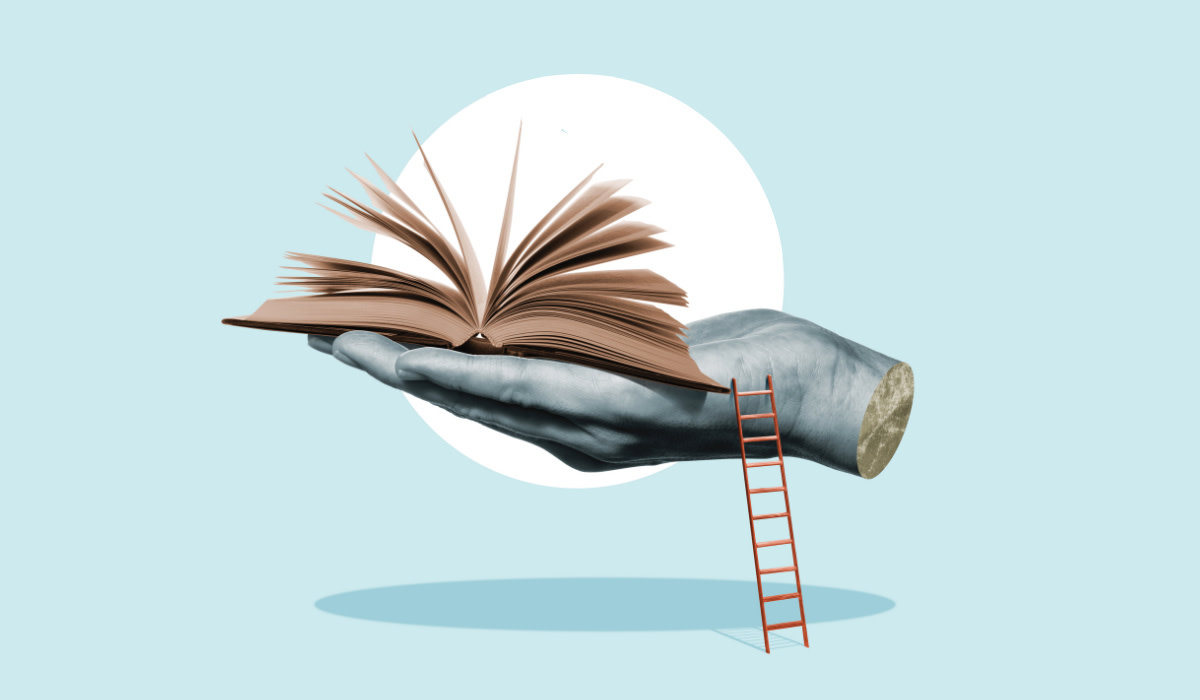
Data cleaning, also called data cleansing or data scrubbing, is the process of preparing data to be stored and analyzed to ensure maximum reliability, validity, and overall quality.
What’s the difference between data analysis and data cleaning?
Data analysis and data cleaning are at opposite ends of the spectrum.
Data analysis involves reviewing and making decisions based on data.
Data cleaning involves ensuring that the data that will be used for decision-making is reliable and of high quality.
Data cleansing, therefore, is an early step in the process that will eventually lead to data analysis.
Talent intelligence involves analyzing and using internal and external data to drive better talent strategies in alignment with organizational goals. HR and company leaders need to have a high level of confidence in their data to trust that the decisions generated are valid.
How is data cleaning used in HR?
People analytics has become a core focus of most HR activities over the past few years as new and more advanced technology has become available. The datafication of HR is the use of analytics to help understand and make better decisions about people, HR processes, and external demographics.
To be most confident in the results obtained from this analysis, it’s important that data is reviewed and cleansed before it’s used to make important decisions. Data quality can be compromised if the data hasn’t been entered properly, if duplication exists, if data is outdated, or if data has come from unreliable sources.
What’s involved in data cleaning?
Data cleaning involves fixing or removing inaccurate, duplicate, incorrectly formatted, corrupted, or incomplete data. Data cleaning processes include finding and removing duplicate entries, fixing structural and formatting discrepancies, and filling data gaps.
The Visier platform consolidates values and data is bucketed into concepts, allowing for more efficient data cleansing and ensuring everything is put into the right file. Subjects and events are linked using a unique instance. Visier’s extract, transform, and load (ETL) workflows remove, replace, and recategorize duplicate records and missing values in your data.
What are some best practices for data cleaning?
Taking a “clean as you go” approach to data cleaning is the most efficient method for organizations to embrace. This allows companies to answer their pressing questions quickly instead of simply focusing on data cleaning for its own sake.
Another important best practice: don’t get caught up in the quest for perfection. There is no such thing as “perfect” data. The good news: you don’t need perfect data to use people analytics to make meaningful business impacts.