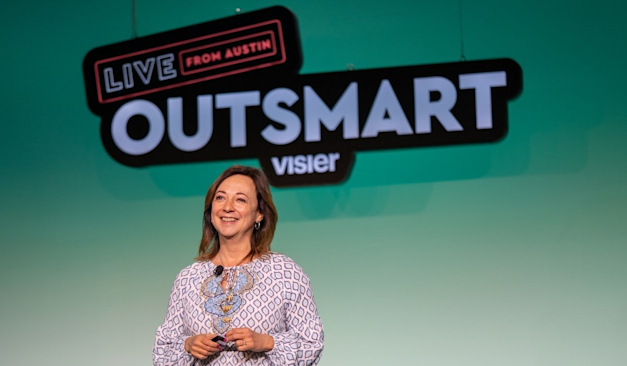
Top Takeaways from Outsmart 2025 ‘Embracing the AI-Driven Workforce'
At the annual customer conference, Outsmart 2025, HR innovators, people leaders, and disruptors gathered for two days of inspiration, innovation, and Workforce AI strategy. Read the top takeaways here.
Latest articles
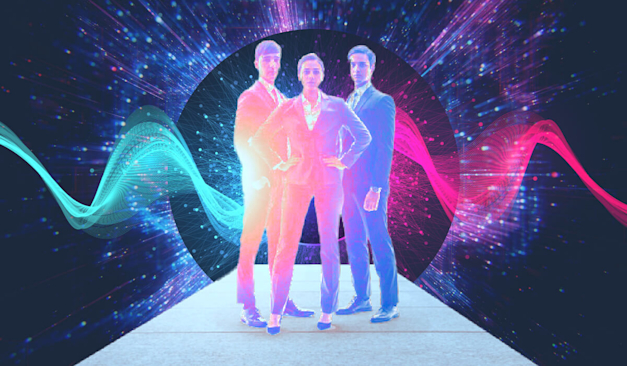
How Generative AI and HR Are Reshaping the Future of Work
To keep up with the future of work, HR leaders need a strategy that's agile, adaptable, and rooted in data. GenAI can help close the gap between people data and business data. Here's how.
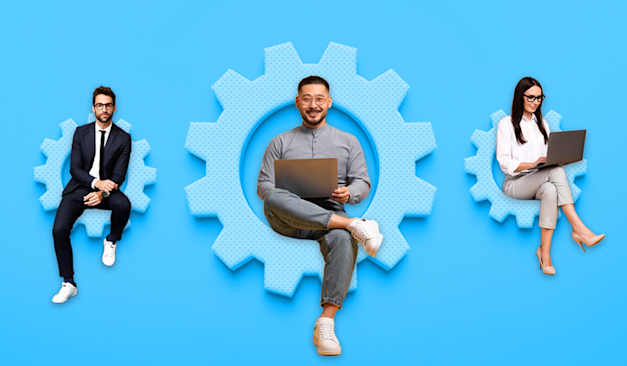
Workforce Optimization in the AI Era: Key Benefits, Strategies, and Examples
Workforce optimization can boost productivity, engagement, and cut costs. Discover key strategies and the power of AI-driven insights. Read more.
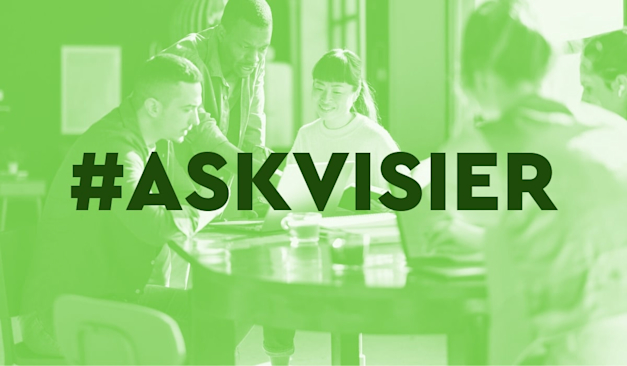
Ask Visier: How can I get started with workforce planning?
An expert shares her thoughts on practical steps to start workforce planning today, leveraging internal capabilities, fostering collaboration, and prioritizing progress over perfection.
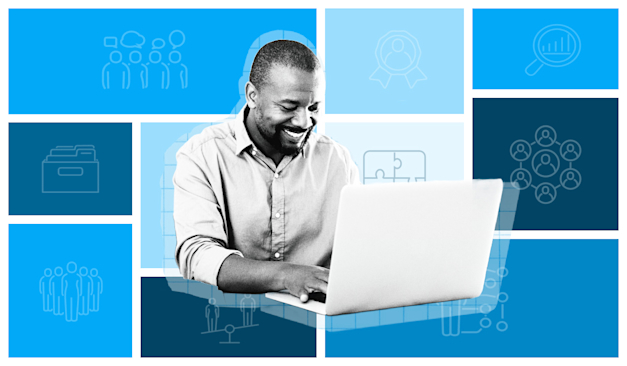
The 10 Essential HR Metrics You Absolutely Need to Watch
HR metrics help you track key workforce activities to improve employee experience and HR effectiveness. Learn the most important HR metrics to track here.
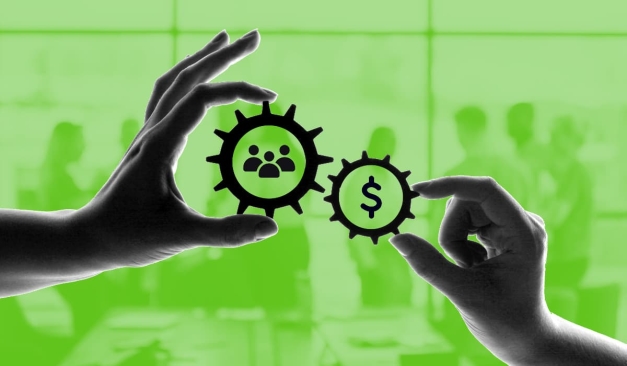
How To Keep HR and Finance in Sync on Headcount Planning
Effective workforce planning requires HR and Finance work as a team. Here's how HR and Finance can keep in sync during three core stages.
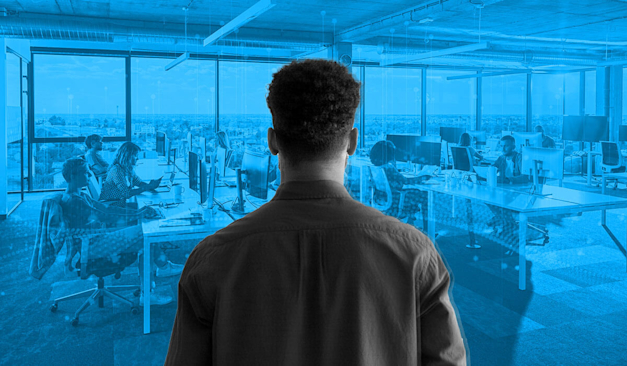
From Analytics to Action: Strategic Workforce Planning in the AI Era
Strategic workforce planning is critical in finding and retaining the right talent and skills to support an organization's short- and long-term goals. Read more to learn what it is and how to do it.
Product & Innovation
Explore the latest in products, the industry, and solutions that bring business data and people data together.
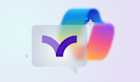
5 Ways To Use Vee With Microsoft Copilot in Your Daily Flow of Work
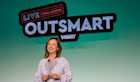
Top Takeaways from Outsmart 2025 ‘Embracing the AI-Driven Workforce'

Introducing Vee Boards, The AI Concierge Analyst for C-Suite Executives
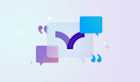
Announcing the Latest Vee Enhancements: Any Question, Any User, Anywhere
All Product & Innovation articlesArtificial Intelligence
We’ve only just begun to scratch the surface of how Artificial Intelligence will change work as we know it.
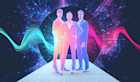
How Generative AI and HR Are Reshaping the Future of Work
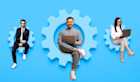
Workforce Optimization in the AI Era: Key Benefits, Strategies, and Examples
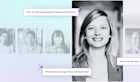
10 Employee Productivity Questions Vee Can Answer Instantly
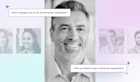
15 Strategic Workforce Planning Questions Vee Can Answer Fast
All AI articles