What Is Data Preprocessing?
Data preprocessing is a series of steps to prepare datasets for analysis and analytics. Learn more about data preprocessing here.
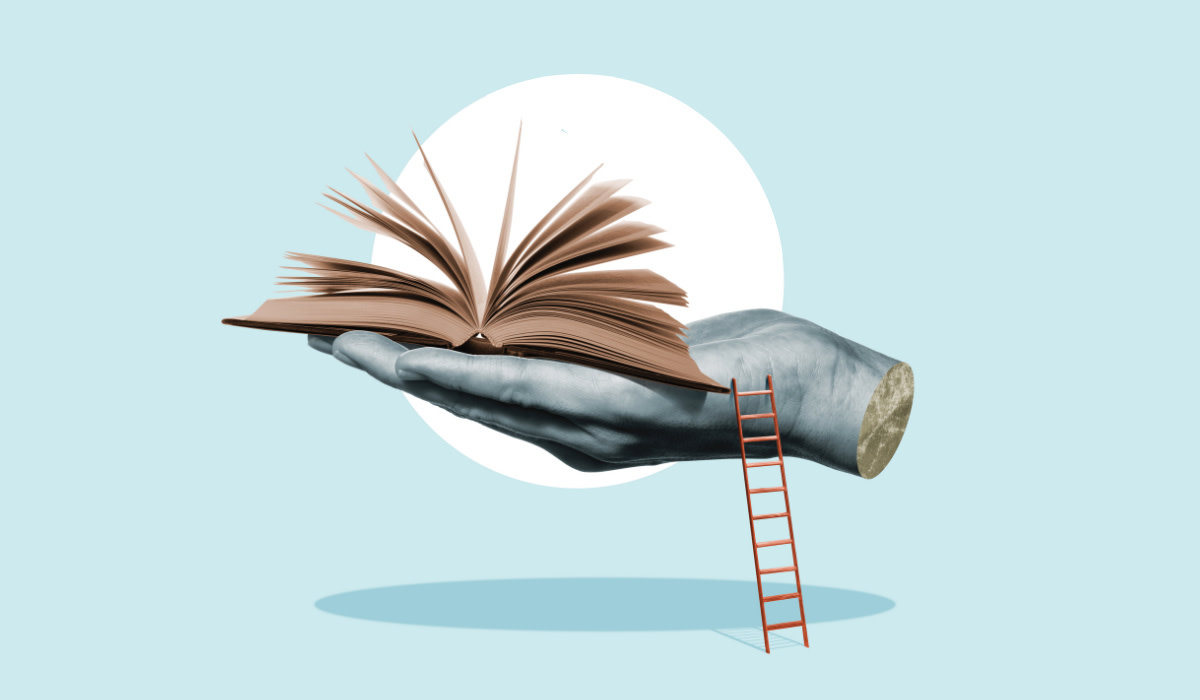
Data preprocessing is a key step in data preparation. It refers to any type of processing that is done to raw data to get it ready for another data procedure.
This is a critical step in preparing for data mining, the process of leveraging the power of big data to generate relevant insights that drive more informed business decisions. As technology has evolved, so has data preprocessing. This technique is now even used to train AI and machine learning models.
Why is data preprocessing important?
One of the greatest potential downfalls related to data analysis is that the data being analyzed is sometimes of low quality. It can be duplicative, contain missing fields or manual input errors, and more. Data preprocessing can help minimize or avoid these types of errors or flaws.
Is data preprocessing the same as data cleaning?
Data preprocessing is not the same as data cleaning, but data cleaning is a part of the data preprocessing process. Cleaning data involves specific steps to detect and correct inaccurate or erroneous records within a specific dataset. This is a key step in data preprocessing.
What are the major steps of data preprocessing?
TechTarget outlines six steps in data preprocessing as:
Data profiling: Examining and analyzing the data and its characteristics.
Data cleansing: Determining how to address data quality issues like eliminating bad data, or adding missing data.
Data reduction: Removing redundant data.
Data transformation: Considering how to best organize the data to achieve the analysis goal.
Data enrichment: This involves feature engineering, or transforming raw data into features that can be used in machine learning models.
Data validation: Here, data is divided into two sets, one to train a deep learning or machine learning model and the other to use for testing.
These steps help ensure that the data you are using for analysis is optimized to minimize potential errors or flawed decisions.
What data preprocessing best practices should we follow?
One of the biggest risks of data analysis is perfectionism. It’s important to not let perfectionism get in the way of progress. While data preprocessing is important, it’s also possible to put your data to work while you clean it. Focus on the key business question you need to answer (like “Do we have a diversity problem?”) and then begin to pull in the relevant data to answer that question.
Read more on data preprocessing:
Get the Outsmart newsletter
You can unsubscribe at any time. For more information, check out Visier's Privacy Statement.