3 Challenges That Can Sink an HR Tech Vendor’s AI Strategy
Product leaders face three major challenges when building and innovating with GenAI. Read more to learn what they are—and how to solve them.
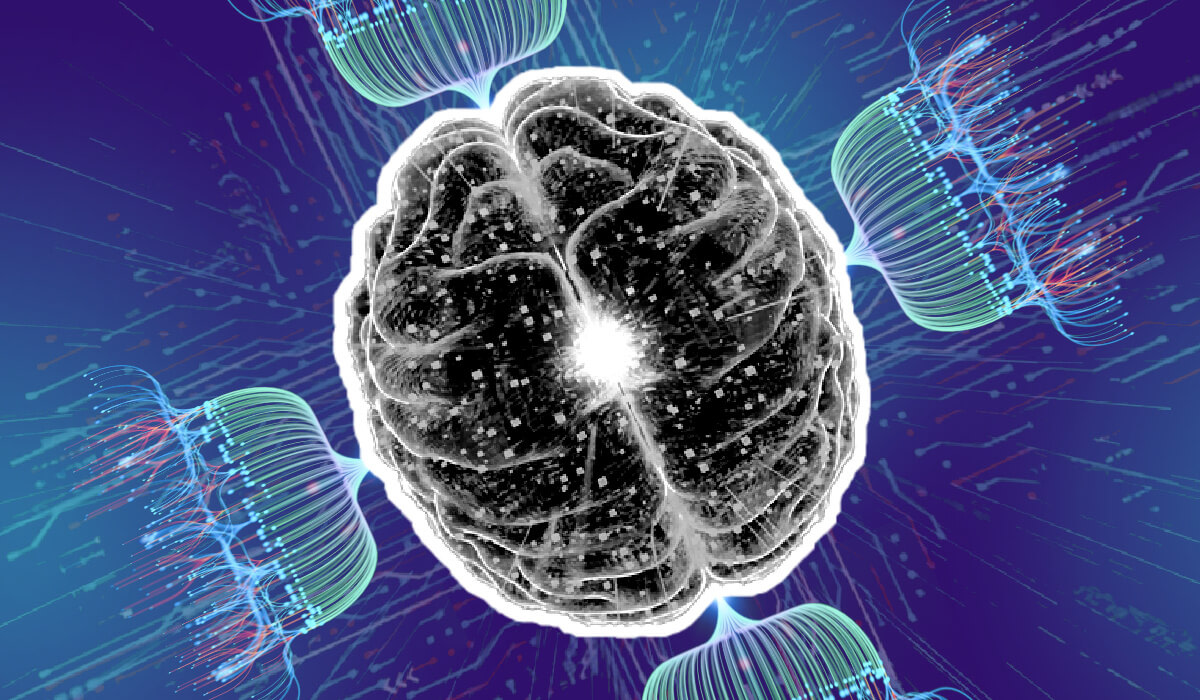
You’re excited about the potential product innovation generative AI can unlock for your organization. As you explore this emerging technology and what to tackle first, you’ll inevitably encounter three challenges, each compounding the next en route to a technological stalemate. Here, we explore three major hurdles that can complicate building generative AI into your product, and offer solutions to help you leapfrog the competition and get you to where you want to go faster.
Challenge 1: The knowledge gap
Let's start with the first challenge: finding the right skills. AI isn’t your traditional technology stack. It demands a deep well of analytics know-how. Plus, it's moving at warp speed. Keeping up with the latest technologies and best practices requires continuous attention and a deep understanding of AI. Progress in regular tech can take months or even years, while generative AI evolution is happening in mere days or weeks.
The problem? This skill set is like a rare gem—hard to find, and even harder to nurture in-house. That's why many product leaders are turning to public, popular pre-built large language models (LLMs). The notion of creating your own equivalent to public LLMs like OpenAI’s ChatGPT and Google’s Bard is exceptionally difficult. Even the open-source community and major players in the game are struggling to keep pace with the rapid advancements made by Google and OpenAI.
Let’s face it. You can’t build your own, and you can’t host your own. You can’t move at the speed of innovation required to keep pace. So, what's the solution? Embrace those publicly available LLMs. Tap into these proven options because acquiring the skill set required to replicate them is just not worth your time and investment. Don’t try to reinvent the wheel at great cost.
Challenge 2: The security dilemma
You’ve recognized leveraging public LLMs is the way to go. Next, it’s time to evaluate security considerations—a topic that's always top of mind. When you opt for public LLMs, you face a new dilemma. These models, clever as they are, lack a crucial feature: the ability to gate responses. In other words, if you train these public LLMs on your data, your data becomes public allowing anyone to query that model for it. There is nothing in it to govern security around the results.
The LLM can’t distinguish between the data access level of one user versus another in order to determine whether the question asker is allowed to know the answer. This is a major security gap when it comes to data as sensitive as people data. You can't give open access to all your sensitive and confidential information in the public domain.
When it comes to HR and people data, there are essentially three layers to this problem:
You don't want the whole world to have access to your data
You don't want your entire workforce to have unrestricted access
You need your employee data to be selectively distributed to the right people, at the right time, in a trustworthy way
And if you cannot find a way to address each one of these layers, then you shouldn’t let your data get anywhere near these public LLM engines, which lands you back at square one.
Challenge 3: The data conundrum
The third challenge is all about data—the lifeblood of AI. Because of the security dilemma, you have to come up with a different security strategy for how to train public LLMs using data. The remaining choice is not to teach the LLM how to respond to your users' questions directly, but instead to teach the LLM how to ask your system the right questions, translated from natural language.
In order to do that, you need to train these LLMs effectively with data that's not just reliable, but also consistently organized. You need to have normalized, well-structured, anonymized, benchmark examples of the questions that people are already asking, instead of your own actual data. Problem is, who actually has significant enough anonymized data on which to train these LLMs? Especially data as nuanced, dynamic, and complex as people data.
This isn't a challenge unique to one organization. It's a hurdle the whole AI community faces. Without well-structured, normalized datasets, your AI aspirations hit a roadblock. The training process, crucial for fine-tuning models, grinds to a halt without this solid foundation. So, it's not just about having data. It's about having the right kind of data.
Vee, the missing puzzle piece
Wondering how we tackle these challenges with Vee, Visier’s new generative AI solution? Well, let’s break it down quickly.
At Visier, we're all about leveraging the publicly available and lightning-fast LLMs. These engines act as an incredibly reliable foundation on which to build new generative AI tools. We stand on the shoulders of giants like Bard and ChatGPT so we can innovate and do what we do best around delivering insights from people data for our customers and partners.
Visier has security and privacy built in from the start with our robust and reliable security model, designed to handle the intricacies of people data with enterprise-grade security, privacy, and user access. We’ve extended this to our generative AI tool as well.
By mapping to the organizational chart, Visier’s platform understands how security and privacy applies to each person asking a question and will adjust the answer based on the role and permissions of the person who is asking—even when asked through a generative AI tool. This is because the gating of responses remains the responsibility of our platform, not the LLM engine. The LLM engine merely helps to translate the question into a specific, relevant empirical data query of the data model. We’ve figured out how to use these LLM engines to ask the right question of your data, but not actually answer the question with your data as the training ground. This brings us to my final point.
Visier has a treasure trove of data—a whopping quarter of a billion benchmark metrics! This means that we don't need to train these public LLMs on sensitive customer data. Instead, we train them using our massive body of data, which includes over 250 million normalized, well-structured, anonymized data points, 2,000 business metrics, and tens of thousands of common people analytics questions.
So when you ask a question using Vee, it understands exactly what you're asking and how to construct the question against our people-centric data model to respond at the speed of thought, delivering back a response that is contextual, accurate, and insightful—all while protecting the confidentiality of sensitive customer data.
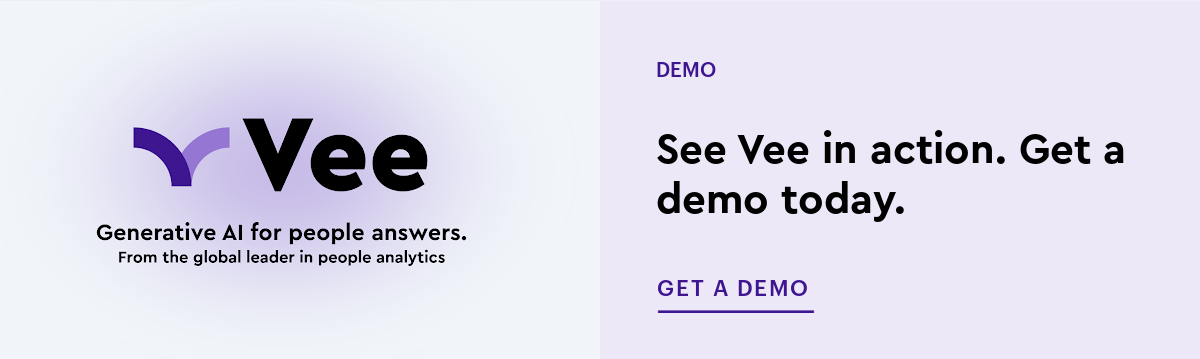
Get the Outsmart newsletter
You can unsubscribe at any time. For more information, check out Visier's Privacy Statement.