Augmented Analytics Explained: What It Is to How To Use It
Augmented analytics helps individuals and organizations extend their ability to collect and analyze data. Learn more here.
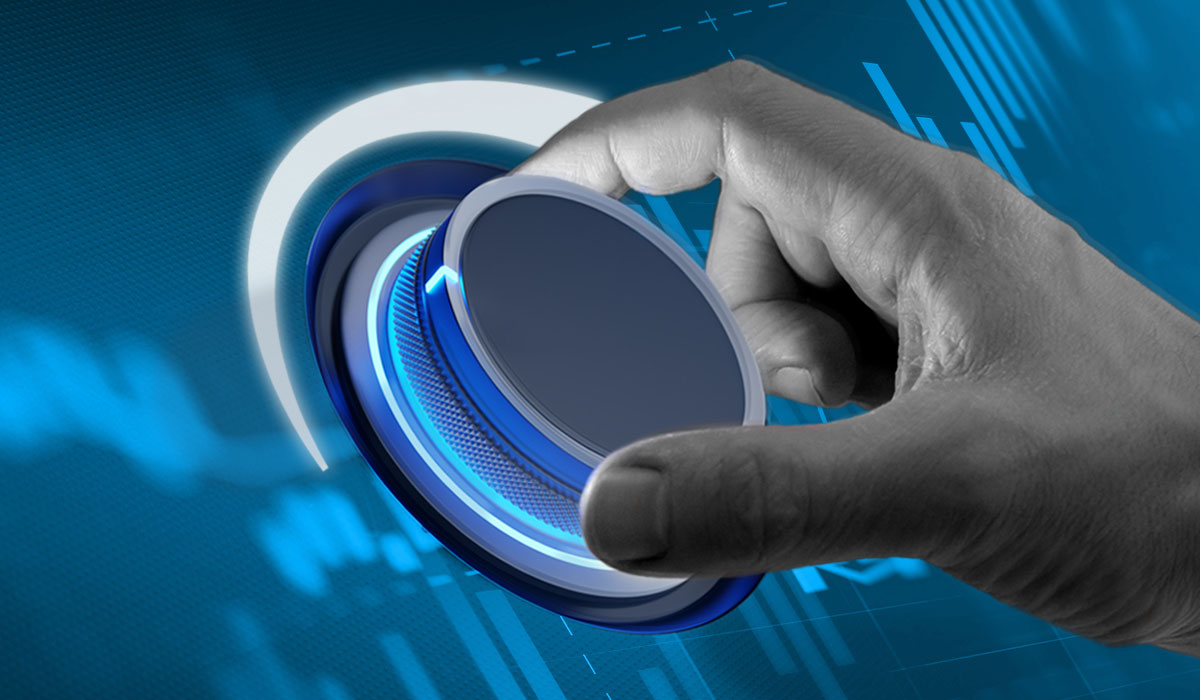
Data is becoming more abundant and complex each day. Difficulties to interpret it arise, and before you know it, you’re making mistakes using faulty information. Augmented analytics uses artificial intelligence and machine learning to improve accuracy and deliver meaningful insights. Here’s everything you need to know about it.
What is augmented analytics?
Augmented analytics uses artificial intelligence (AI) and machine learning (ML) to collect and analyze data and provide meaningful insights.
Don’t confuse it with AI analytics, though. They are somewhat similar, but they have different purposes. AI analytics uses artificial intelligence techniques to analyze data. It has a higher level of complexity and is suitable for data scientists and analysts.
Augmented analytics, on the other hand, simplifies the data analysis process, which makes it a great tool for non-analytics experts. It can be a tool for HR and business leaders, helping them gain meaningful insights from their data.
Key components of augmented analytics
Augmented analytics has three key components: machine learning, natural language technologies, and automation. Let’s look at each one in more detail.
1. Machine learning
Machine learning (ML) leverages algorithms that “learn” by parsing large amounts of data. It is a field of AI and can be used to identify patterns and trends.
ML algorithms thrive when they continuously learn from both structured and unstructured data. The more data you have, the more accurate these algorithms become. Within seconds, they can spot trends and patterns that would often be impossible to notice with the naked eye and traditional analysis tools.
2. Natural language technologies
Natural language technologies make human-machine communication easier. Natural language processing (NLP) helps computers interpret human language.
Natural language generation (NLG) turns computer code into a natural language that humans can understand. Together, they make data analysis easier even for those without a deep knowledge of data science.
3. Automation
The final, crucial component of augmented analytics is automation. Without it, data scientists would have to spend hours or even days going through data trying to gather insights.
ML-driven technologies can automate these processes, making it easier for users to discover and analyze data. As a result, you will spend less time on technical tasks, and more on creating data-driven business strategies.
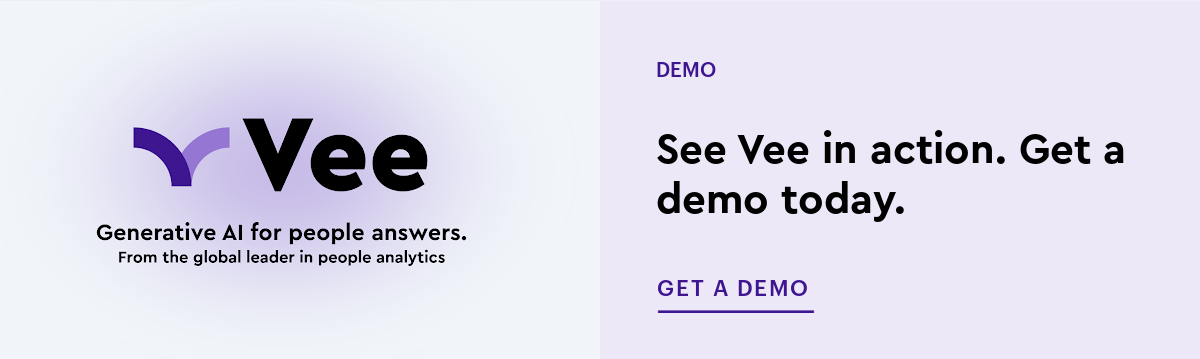
5 key benefits of augmented analytics
Augmented analytics is an essential tool for businesses that want to leverage the power of their data effectively. Here are some of its benefits.
1. Improved decision-making
Augmented analytics helps you make better, data-driven decision-making. With faster and more accurate analysis, you can focus on the data that matters, creating business plans, setting goals, and improving processes to reach your goals faster.
2. Reduced bias
No matter what you do, human bias is a real issue that can make its way into your decision-making process. You may not even be aware of it until it is too late. Augmented analytics help you reduce both conscious and unconscious biases.
By relying on data and algorithms, you can be truly objective and make decisions that benefit not just the business, but also your employees and customers.
3. Faster insights
Augmented analytics, and specifically the use of ML algorithms, help you streamline data collection, preparation, and processing practices. This makes the entire process faster. Plus, the algorithms will be quicker in identifying patterns, trends, and even anomalies, helping you make the most of your data and find the insights you need.
4. Higher accuracy
Algorithms can also be wrong, and that is true for AI and ML. But the more users interact with these tools, and the more data they provide, the higher their accuracy.
Plus, in augmented analytics, the machines aren’t doing all the work. People will analyze the results, accepting or rejecting the machine’s suggestions. This entire process will lead to higher accuracy in data interpretation, reducing both human and machine errors.
5. Improved efficiency
Augmented analytics help you automate a lot of your data-related tasks. Collection and analysis become easier and faster and require less and less intervention as time goes on.
As a result, analyzing data becomes more efficient and you can spend time on other tasks, such as gathering insights, creating business plans, and improving business processes.
5 challenges of augmented analytics
Augmented analytics is an invaluable tool for businesses across multiple sectors. But using it is not without challenges. Here are some of the most common ones you’ll face.
Integration challenges. Integrating augmented analytics with your existing tools is not always easy. Depending on your systems, you might need to spend a lot of resources to get everything running smoothly.
Privacy and security. ML and AI algorithms learn from data. But using private information with augmented analytics comes with privacy and security risks you must first consider. Ensure you have permission to use the data and that you’re compliant with any privacy regulations in place.
Potential bias. Machines can be biased too. This happens when ML algorithms learn from faulty data sets and lack of context. To avoid this, make sure you use accurate data and choose tools that have context-aware capabilities.
Irrelevant results. The insights provided by augmented analytics algorithms won’t always be relevant. That may come from poor prompts or data sets that are too large and contain irrelevant information. Ensure the software you use can filter out the information that’s not useful so that the insights can truly benefit the users.
Scalability challenges. Augmented analytics thrives on large quantities of data. Unfortunately, not all IT systems have the necessary computing power. Plus, increasing the volume of data may slow down the response time.
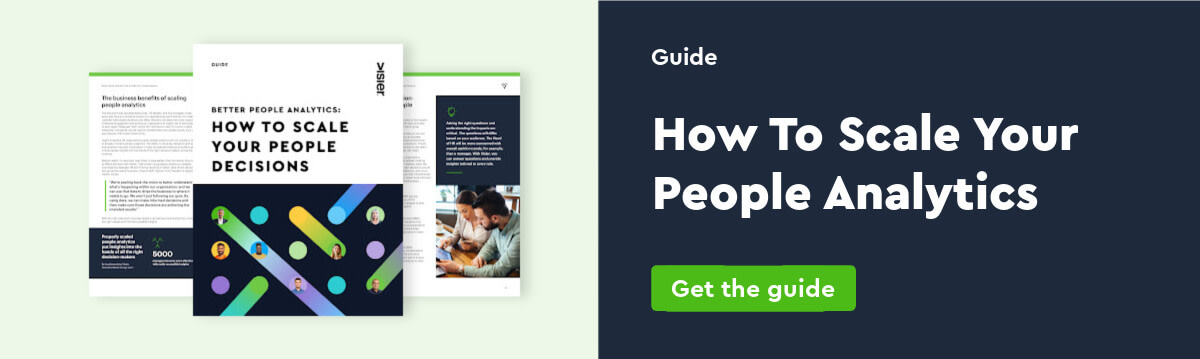
Augmented analytics use cases and examples
You can use augmented analytics in almost all business sectors. From sales to HR, everyone can benefit from its insights. It is also a valuable tool across various industries. You can use it in IT, but also in hospitality, marketing agencies, and supply chain management. Let’s take a look at some real-world examples.
HR talent management. HR teams can use augmented analytics to identify and retain top talent. They can also look at key HR metrics and see which top performers are at risk of leaving soon. With this information, they can create targeted retention programs to decrease voluntary turnover.
Sales forecasting. The sales department can use augmented analytics alongside predictive analytics. They can predict future sales trends using historical data, information regarding demographics, economic indicators, or seasonality.
Financial fraud detection. Banks and other financial institutions can use augmented analytics to improve fraud detection systems. By analyzing transaction data and patterns, you can identify unusual activities and anomalies, preventing fraud and financial loss.
Supply chain management. Augmented analytics can help improve supply chain organization. For instance, you can understand why certain locations have a delivery rate below expectations. You can also forecast supply demands and create more effective supply chain processes.
Best practices for using augmented analytics
When you use them correctly, augmented analytics can help you reach business goals and improve various processes. Here are a few best practices to help you on your journey.
1. Start small
You want to do everything as quickly as possible. That’s natural, but you may need to slow down at first. Trying to implement augmented analytics across too many sectors at the same time can lead to more issues than benefits.
Start with a few smaller goals and pay close attention to their related metrics. See how the implementation goes, and how much improvement it brings. If you’re unhappy with the results, make adjustments and repeat. When you’re successful, you can move on to larger projects.
2. Offer training and support
While non-specialized users can use augmented analytics, some may still find it challenging. With any new concept, there is a learning curve.
How successful you are with implementing augmented analytics will largely depend on how well you support your employees through the learning process.
Plus, some people are still skeptical regarding anything AI-related. A custom training program can help you improve AI and data literacy and build trust.
3. Ensure data quality
Augmented analytics is only as good as the data it works with. If your data sets are inaccurate or outdated, your results will be far from optimal. Invest in regular data checks to make sure you’re using reliable data, free of errors.
4. Encourage collaboration
Many users feel AI lacks transparency. Invite everyone to collaborate on the project, so that they can better understand how you’re using augmented analytics.
This doesn’t mean every employee needs to interpret data. It can be as simple as including them in learning and development programs that will help them gain understanding and trust towards the use of AI and ML algorithms.
5. Don’t forget about regulatory compliance
Last, but not least, keep an eye on data privacy regulations. Some jurisdictions have stricter rules, especially when talking about automated data processing and using AI. Make sure you’re respectful of everyone’s privacy, ensure data security, and comply with the regulations that apply to your business.
Augmented analytics is a powerful tool that can change the way you look at your data. It can improve your decision-making process, helping you rely on objective, accurate data. It can eliminate biases and improve efficiency across various business sectors. Use the data to understand what drives your employees, how to improve various aspects of your business, and reach your business goals faster.
Want to see how easy it is to set up self-serve people analytics at your organization? Start a 30-day free trial of Visier People®, which comes with sample data and complimentary access to email and community support to help you get up and running—fast.
On the Outsmart blog, we write about workforce-related topics like what makes a good manager, how to reduce employee turnover, and reskilling employees. We also report on trending topics like artificial intelligence, using generative AI in HR, and how skills are rapidly evolving, and advise on HR best practices like how to create a strategic compensation strategy, how to manage HR data, and how to use reports vs. analytics. But if you really want to know the bread and butter of Visier, read our post about the benefits of people analytics.

Get the Outsmart newsletter
You can unsubscribe at any time. For more information, check out Visier's Privacy Statement.