3 Ways to Neutralize AI Bias in Recruiting
In this article, discover three tips to leverage AI in support of your diversity goals and ensure AI neutralizes–and does not perpetuate–human bias.
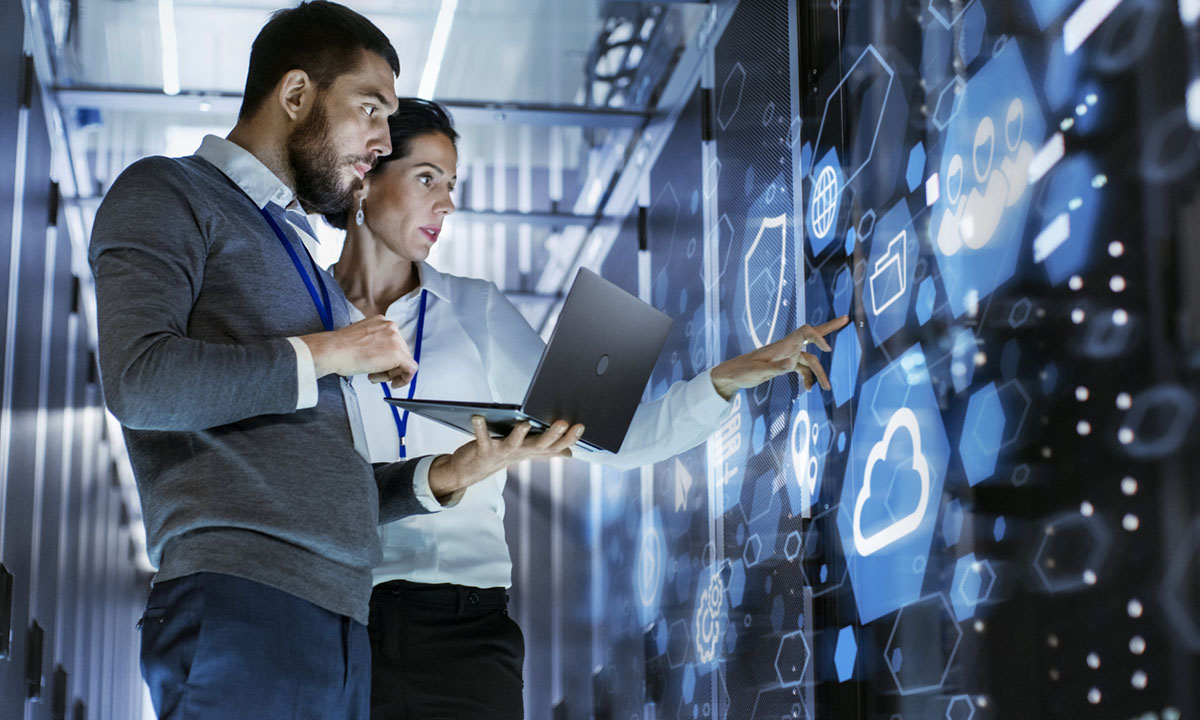
If there is one thing to learn from Amazon’s experience with a sexist recruiting engine, it’s that AI is a terrible master — and perhaps, it is better suited for the servant role.
About three years ago, the company’s machine learning specialists noticed something unsettling about the experimental recruiting algorithm: Having learned from the training data that male candidates had more experience in technical roles, it started penalizing resumes that included the word “women’s” as in “women’s volleyball.” It also downgraded graduates of two all-women’s colleges.
Simply put, the algorithm had learned to put women at a disadvantage. As a result, the project was scrapped, and the company never used the AI application for actual hiring situations. (Kudos to Amazon for going through this validation and not rushing to deployment.)
This is certainly not the only episode of algorithmic bias. Months before Amazon’s AI story was leaked to the press, experts speaking on a panel at an MIT event warned that the algorithmic revolution in hiring was moving too fast. AI or machine learning (a branch of AI) needs to be trained to complete tasks, and any application that is trained on data containing bias will replicate that bias.
For all its limitations, however, AI should not be overlooked. The melding of neuroscience and AI has produced some positive results in terms of helping organizations level the playing field for women and minorities. AI is also showing promise in terms of helping organizations attract more women to technical jobs.
Neutralizing Human and Algorithmic Bias to Boost Diversity
Diversity and inclusion is now a business imperative, and research shows that even well-intentioned recruiters are prone to implicit bias. One intensive academic study revealed that minority applicants who “whitened” their resumes were more than twice as likely to receive calls for interviews, and it did not matter whether the organization claimed to value diversity or not. When it comes to fairly assessing job candidates, humans could use quite a bit of help.
To ensure AI neutralizes — and does not perpetuate — human bias, HR leaders need to consider three things: the nature of their organization’s diversity problem, evidence of success in using AI to overcome specific challenges, and vendor conscientiousness.
Use these three tips to leverage AI in support of your diversity goals:
#1. Point the Technology at the Right Problem
AI has been touted as a way to help recruiters sort through a sea of resumes more efficiently. Getting an AI tool to help your recruiters wade through resumes at a faster rate, however, may not be the best place to start if not enough women or minorities are applying for open positions in the first place. In other words, sorting resumes faster is irrelevant if you do not have enough female candidates!
For example, research reveals that females tend not to apply for roles that sound too masculine. Organizations have zoned in on this issue by using Textio (an augmented writing platform that uses AI to match the words used in job descriptions with hiring outcomes) to write gender-neutral job ads. Here, the algorithm is looking for common, well-understood patterns on which it has been trained and replacing them with properly-crafted alternatives.
As the global head of diversity at Atlassian told CNBC, Textio is one of the main interventions that helped her company boost its hiring of women for technical roles. By targeting the problem — self-selection of female candidates — the company was able to find the right solution.
So before rushing to adopt AI in support of diversity, look at all stages in the recruitment pipeline, from applicant volumes to hire rates, and determine where you are falling short of your diversity goals. You will then be in a better to position to assess where you need to intervene so that you can move the needle on outcomes.
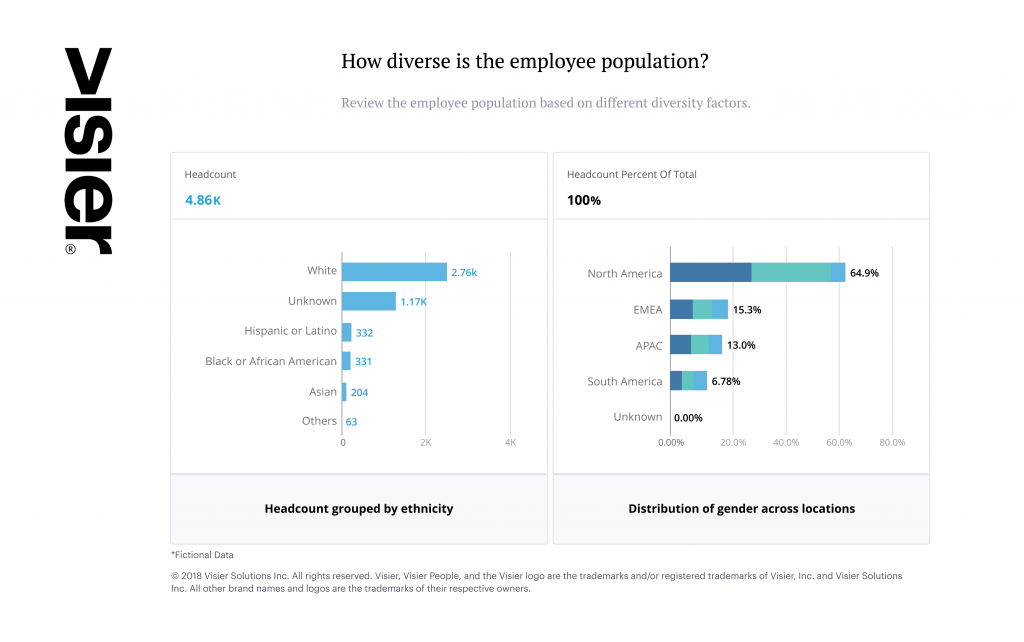
Data visualization showing the breakdown of diversity in a fictional organization’s workforce
#2. Boost Diversity With Proven Approaches
It can be easy to claim that AI will help combat bias, but not so easy to prove it. HR leaders must look for evidence that a particular approach has successfully helped an organization achieve its diversity goals before making the leap.
One area where companies are successfully leveraging AI is in the realm of candidate assessment. At Unilever, candidates apply for jobs via LinkedIn, and then play neuroscience-based games that measure cognitive and behavioral traits on a platform provided by technology company Pymetrics. The results are analyzed by algorithms that compare an applicant’s skills with those of employees.
Since implementing this approach, reports Business Insider, the company has experienced a significant increase in hires of non-white candidates.
One reason for this success may be due to the fact that the technology is new, but the approach is not. There was a phase in the 90s where assessment centers were commonly used. Candidates would perform tasks for two days while observed by expert psychologists. This did produce robust and unbiased results, but it was expensive and typically reserved for executive positions. With AI and other technologies, this kind of assessment can be delivered at scale.
#3. Select Vendors Who Have a Handle on Algorithmic Bias
When purchasing AI software, it is important to work with your technical peers to make sure the vendor understands what kind of interventions are required to address algorithmic bias.
For example, the software can be programmed to ignore information such as gender, race, and age. At Visier, we leverage machine learning in our risk of exit models, where we can choose to input or not input certain properties related to race or gender. By removing these properties from the model, we can mitigate the risk of bias creeping in.
Conscientious vendors also continuously share the validation results for their algorithms. These validations will highlight whether or not results are introducing bias. However, as the underlying math becomes more complex, testing, and then controlling all the outputs becomes more challenging. Amazon’s algorithm, for example, was working with very complex data inputs that contained “shades of grey” references to gender (i.e. “women’s chess club”). This is far more challenging to control for than a simple statement of gender (i.e. male/female).
At the end of the day, before putting AI in the field, HR leaders need to ensure they are selecting technology that has been — and will continue to be — properly tested and validated.
AI: The Human in the Loop
The biggest distinction between human judgment and AI is that algorithms are binary or probabilistic — ethics is not. That being said, there may come a day when AI systems can make ethical decisions. Experts have predicted that AI will be better than humans at “more or less everything” in about 45 years. In the meantime, though, we will need humans in the loop. This way, AI systems can help serve, not rule, human decision-making — and where we need the most help, it seems, is in the judgments we make about other people.