Workforce Analytics: Everything You Need to Know
Learn how to improve workforce management, planning and business outcomes with workforce analytics. Read more.
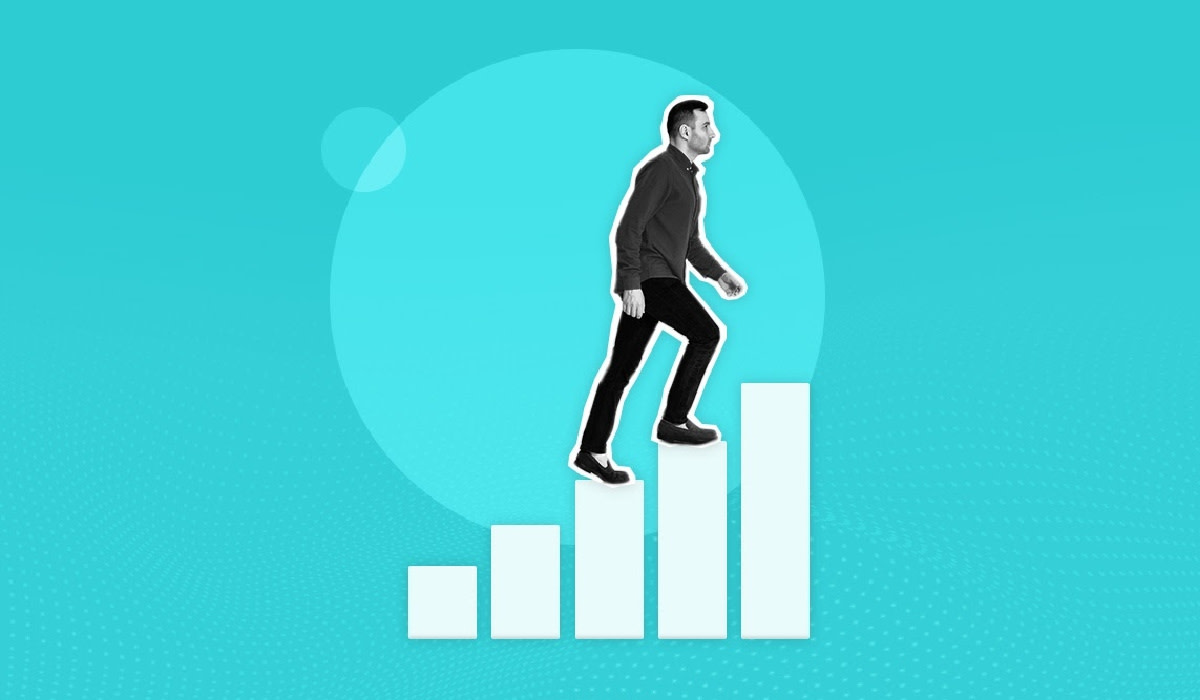
When you start a business, it’s likely you’ll have very few employees. But as your business grows, so does its complexity. Workforce analytics is a set of tools that helps you manage employee data. They can also aid in boosting employee performance, reducing absenteeism, and more.
By using workforce analytics you can create an environment where employees feel valued. Along with people strategies and HR analytics, it can reduce turnover and help you reach your business goals.
What is workforce analytics?
Workforce analytics is a combination of data-informed methods and tools that aim to measure and improve workforce management and planning.
Data is collected from both internal and external sources. The analysis is then turned into actionable information.
The goal is to measure how workforce behavior impacts business performance.
What is the difference between workforce analytics and HR analytics?
HR analytics is an analysis performed by the HR department. It is also known as staff statistics, demographics, or talent statistics. It is a way of analyzing and managing employee data.
Workforce analytics is not as focused on human resources data. Instead, it takes a broader approach to work data. Sure, people are part of it. But they’re not the only element that matters.
What is workforce analytics used for?
Workforce analytics helps review things that were done in the past and measure the results. It can also help predict future behavior and results. Finally, it can be used in the decision-making process to improve workforce planning and management.
Workforce analytics can be applied to areas like:
Recruitment;
Talent management.
The benefits include:
Identifying and recruiting people who are aligned with the company’s culture and its needs;
Identifying issues that may increase turnover. This includes lack of promotion opportunities, too little or no training, or discrimination;
Better employee experience;
Predicting turnover and required workforce in the future;
Analyzing and understanding which recruitment tactics are the most effective;
Understanding and reducing employee absenteeism;
Improving employee performance.
5 steps for implementing workforce analytics
Implementing workforce analytics doesn’t have to be complicated. You just need a clear vision and an openness to change. Here are five steps that will help you implement successful workforce analytics.
1. Determine your intention
You can’t start any new project without a clear intention. And workforce analytics is no exception.
Maybe you want to have better employee retention. Or maybe want to recruit the right people faster, and more effectively. Maybe you’d like to improve retention or the overall employee experience. Whatever your goals are, be very clear about them.
Write your goals and any other evidence you have at this point in an official project document. It makes it easy to visualize your intention and keep track of your steps.
The document will also be easy to share with your team. After all, you need to make sure everyone involved in the analytics process is working towards a common goal.
2. Gather data
Now that you know where you’re headed and why it’s time to gather the data. Based on your goals, you and your team can establish which workforce data will be most helpful.
There are several ways to collect information which include, but aren’t limited to:
Manual data collection;
Employee surveys;
Automated software.
Don’t forget about the ethical and legal factors, data privacy, and security. Depending on where your organization is located, you might need to pay attention to local data processing laws.
Finally, when gathering data, don’t limit yourself to HR resources. Accounting and various project data can provide a good idea of workforce behavior.
3. Build your workforce analytics dashboard
A workforce analytics dashboard will help you visualize data in real-time. There are various software tools that can help you do this. But if you don’t want to invest or learn to use a new tool, spreadsheets, and other visualization tools can be enough.
A lot depends on the amount of data you’re gathering and how you interact with the rest of your team. Choose something that makes the process easy and quick.
4. Analyze the results
You’ve got your data, and a dashboard, it’s time to compare and analyze the results. During the first two steps, you should have defined an ideal benchmark and objective.
How are your results compared to that? What do the results tell you about your workforce?
These answers will help you define your strategies going forward.
5. Report the results
The final step is creating your report. To do so, be sure to answer the following questions as clearly as possible.
Have you met your goals?
What new information did you learn through this project?
What changes should you be making based on the data you found?
What can future goals look like based on where you ’re at right now?
The report can also be presented to your employees so they can understand what you’re expecting of them and how they can help.
3 examples of workforce analytics in practice
We talked about what workforce analytics is and how to do it. But what does it look like in practice? Here are a few examples of how this practice can help a company. As a bonus, we added a report by the Enid Regional Development Alliance that shows the power of workforce analytics.
1. Recruitment
A bad hire can cost over $200,000. So investing in things that can help you avoid that makes sense.
A way to use workforce analytics to help you in the recruitment process is the use of surveys. Next time you’re hiring, provide candidates with a series of questions to understand them better.
Don’t limit yourself to things like academic background or experience. Try to learn about preferences like benefits, diversity, or career growth opportunities.
Exploring the market and your competitors is another way to use people data to your advantage. A company that is well-known to use such analytics tools for its recruitment process is Reece Group.
The goal is to know and understand your candidates so that you can make the best choice possible.
2. Employee management
How are your employees performing? How is that performance impacting your business, sales, and customer experience? What factors can help improve overall business results?
In short, what factors related to your employees have the most impact on your business?
Workforce analytics can provide many of these answers. Collect data relating to personality traits, interaction with customers, behavior, and more.
Compare this data against things like financial incentives or learning and development opportunities. You can also consider other factors such as length of shift or coworker collaboration. Does a relationship exist? What helps people perform better?
3. Retention
A high voluntary turnover rate can have a negative impact on business performance. And it can cost you a lot of money. So what helps you retain people?
Analyze data pertaining to things like:
Recruitment;
Performance;
Experience;
Salary;
Tenure;
Job Role;
Location.
Engagement with other employees and growth opportunities can also be added to the analysis.
What do the results tell you? Do you see any factors that indicate a person may be preparing to leave soon?
Results vary from company to company. Often, the main culprits seem to be a lack of engagement or few to no growth opportunities.
Bonus — Sample workforce analytics report
The Enid Regional Development Alliance published its workforce analytics report in 2020. Their analysis looked at things like demographics, workforce training, and occupational dynamics. They compared their data against market competitors to ensure their results were accurate.
The analysis helped them identify key strengths, challenges, and opportunities across various sectors like production, maintenance, healthcare, and more.
Workforce analytics FAQ
What is the difference between workforce analytics and people analytics?
People analytics only looks at data relating to the people in the company. Workforce analytics looks at data pertaining to work in general. People still play an important role, but they’re not the only component that matters.
Who uses workforce analytics software?
Workforce analytics software is usually housed in the HR department. But it is not only available to those working here. It can be made available to executives, managers, and other employees. In most cases, there are different permission levels. A custom vision dashboard might also be available to each access level.
How can workforce analytics help organizational teams other than HR?
Workforce analytics can help any department understand turnover rates, productivity, and other such trends. As a result, teams can focus their efforts in a direction that will help improve the organization’s processes.