3 Ways People Analytics Ends Employee Retention Guesswork
Discover key employee retention analytics HR leaders need to track when tackling turnover and building powerful retention programs.
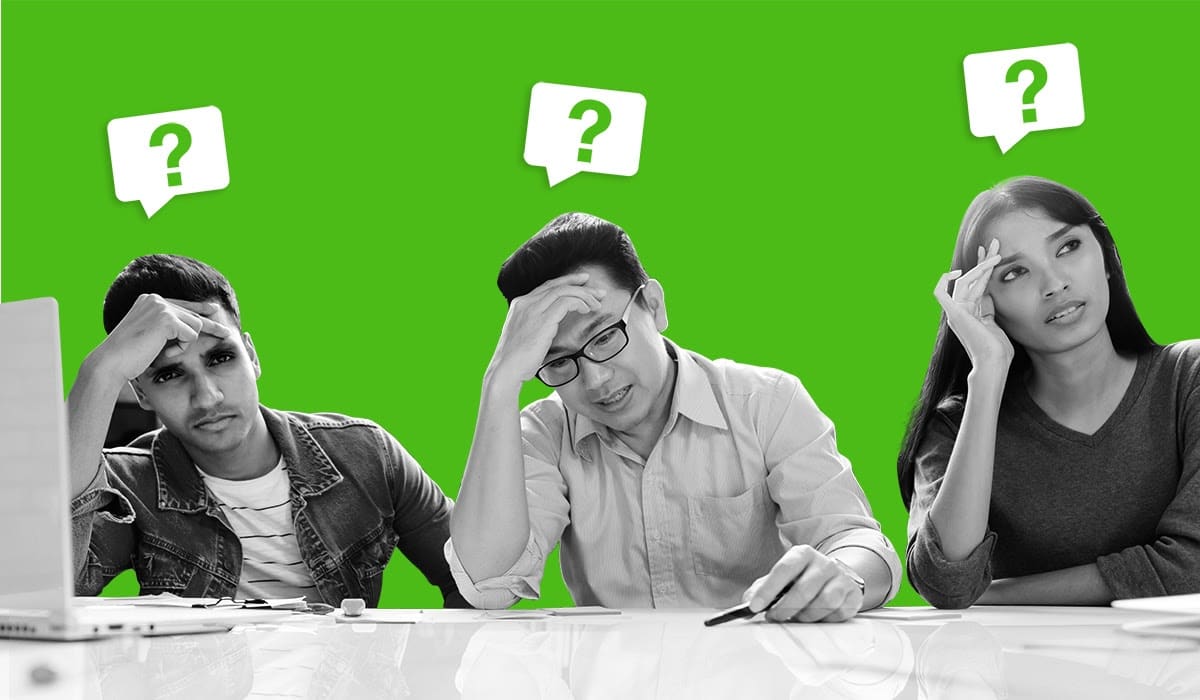
In this article, discover key employee retention analytics HR leaders need to track when tackling turnover, and how people analytics can help uncover the relevant insights to build powerful retention programs.
It turns out that HR shares a passion with economists. Hiring, vacancies and turnover are critical measures on both the state of the economy and the health of the organization.
If you read the financial papers, economists will point to record levels of new job openings, and what follows — voluntary resignations. While most organizations will focus on improving their ability to hire, the savvy ones know that retaining talent is just as critical. Some turnover is healthy for organizations, but the danger in such record-setting times is passing a critical threshold of talent loss.
Every organization has the resiliency to absorb the loss of talent, but that resiliency has a limit before serious business impacts start occurring. Identifying the why, where and who of flight risks before they appear is the only way to proactively avoid losses in productivity, revenue and profits.
For many organizations, voluntary turnover costs millions of dollars. When the direct costs of replacing an employee, interim reduction in labor costs, and costs of lost productivity are all taken into account, the total cost of voluntary turnover is $109,676 per exiting employee for an average U.S. organization, according to Bersin by Deloitte research.
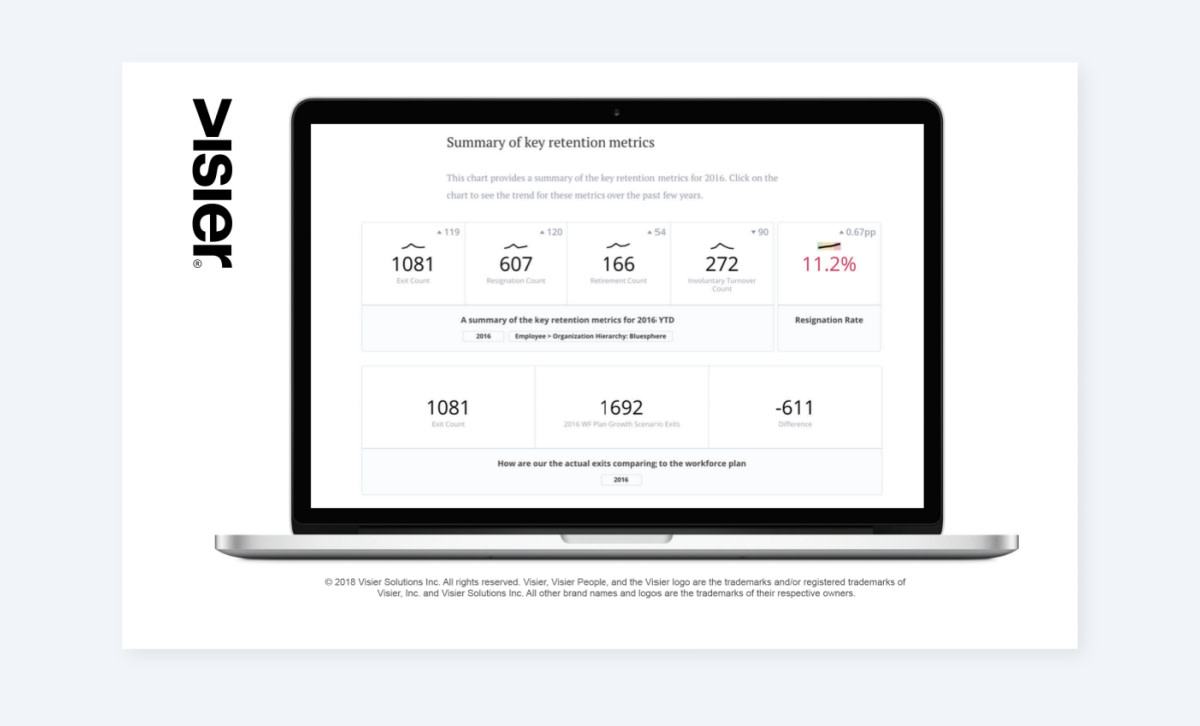
But when well-intended employee retention tactics are applied to everyone in the organization, their results can be limited, doing little to alleviate flight risk. We would never consider a “peanut butter” strategy for compensation adjustments, but retention programs are too often spread thin across the organization, which increases costs and decreases effectiveness.
The answer? Rather than crafting across-the-board retention programs, HR organizations need to sharpen their focus and apply dollars where they’ll have the most impact. This requires businesses to be far more sophisticated in uncovering the root causes of turnover, and to adopt more analytical approaches that increase their ability to identify flight risk factors and arm business partners with the data to drive action.
Here are three key questions HR leaders need to ask when tackling turnover and how analytics can help uncover the relevant insights:
Question #1: Where is the greatest business risk?
Before designing retention initiatives, you must first figure out the impact of churn. To determine this, drill down to understand the consequences of losing talent in different departments, roles, functions or geographies. After all, what counts as high turnover in one area may be normal in another. Ultimately, the greatest business risk exists where exceptionally high turnover exists for talent with high impact to business outcomes.
For example, the people analytics team at BBVA USA, a U.S. banking franchise, discovered that turnover was highest for one particular revenue-producing role. By using an analytics platform, they quickly discovered that at certain branches, turnover for that role was significantly higher: 70 branches — about 10 percent of all branches — accounted for 41 percent of all turnover.
To help improve retention, the team crafted a strategy targeting the 70 high-turnover branches. The results have been significant: Annual turnover for the revenue-producing role has improved dramatically at those branches, dropping by 44 percent since the start of the project.
Another organization, Children’s Health, used analytics to build a compelling business case that supported the implementation of programs to reduce turnover. This included a detailed cost-of-turnover analysis with a bottom line of almost $15 million of projected savings over three years.
Drilling into the data, the HR team discovered that some hospitals had a higher than average first-year turnover rate. After examining onboarding practices and other infrastructure, they focused on several key initiatives, such as pay. It turned out that a very slight increase in hourly wages was a powerful retention tool, and far less expensive than what the organization was incurring with chronic turnover costs.
Thanks to these programs, the HR team was able to drive down first-year voluntary turnover from 17.8 percent to 15.3 percent.
As these examples show, by pinpointing where the problem is happening and the impact to the business, HR can strategically invest in programs that will have the biggest results.
Question #2: Why are people leaving? Why are they staying?
To tackle flight risk effectively, it’s also important to uncover the why of the turnover problem.
Micron, a world leader in memory technology, focused on creating clearer job descriptions after it discovered that workers were more likely to leave if they felt their job hadn’t been accurately described when they were hired.
For other companies, the solution involves a greater emphasis on hiring from within the community (if relocation is an issue) or helping employees establish social ties at work (if job embeddedness is the problem).
The reasons for turnover vary, and identifying the key drivers of resignations allows HR to develop refined retention programs by targeting root causes.
Machine-learning techniques can scan hundreds of employee attributes to uncover hidden patterns behind increasing, or decreasing, resignations. Trained on the thousands of historical resignations, these advanced analytical approaches often find the patterns that humans miss: Unsupportive managers, excessive commute times, lack of training, poor onboarding and other factors may be difficult for leaders to observe.
With an analytics platform that pulls together records from the HRIS, talent management, applicant tracking, engagement, learning management and other HR systems, and connects the data, the patterns subsequently uncovered can span the employee lifecycle. The processes of hiring, performance management, compensation and training shouldn’t exist in silos, and neither should analysis of the data these processes collect.
Question #3: Who exactly is likely to leave?
Ultimately, machine learning algorithms that bring data together across the employee lifecycle can go beyond finding the patterns of why people are leaving, and make predictions about who presents the greatest flight risk. Knowing who is likely to leave is incredibly important, because it allows you to make the most targeted action plans to retain talent. Individual retention plans can be created for key talent that addresses factors personal to them.
Predicting flight risk can involve some pretty sophisticated technology and techniques, but it need not be rocket science: Ultimately, it’s about building a profile of the organization’s talent who left in the past, which can then be used to identify similar characteristics in existing employees.
Advanced “in-memory” people analytics applications make it easy to run tailored algorithms to help identify flight risk. Many predictive technologies are based on simple regressions or static models, instead of machine learning which involves continual adaptation and refinement.
One particular machine learning technique, called “random forests,” examines historical employee data and employee events like promotions, resignations and internal hires to learn a set of patterns and construct decision trees that help predict the occurrence of an event. This has been proven by data scientists to be up to 17 times more accurate than other methods.
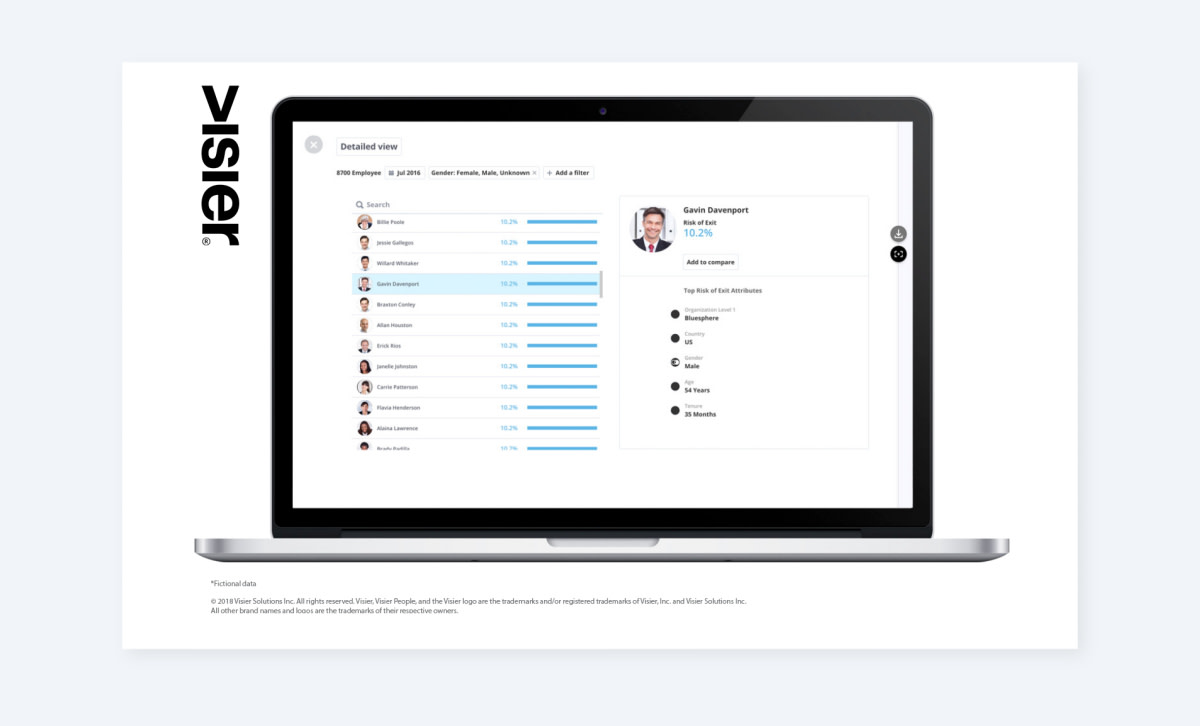
The end of retention guesswork
“We see some problems as just part of the fabric of everyday life, inevitable and immutable, and miss spotting the potential to do something about them,” writes business journalist Amanda Lang in her book, The Power of Why. High levels of attrition need not be accepted as a fact of life.
Armed with workforce data, HR can tackle turnover with a razor-sharp focus on the issues at hand. A more data-driven approach makes it easier to quantify the impact of key programs, helping HR garner the kind of executive support that is crucial to retention success.
This post first appeared on Recruiting Trends.
Get the Outsmart newsletter
You can unsubscribe at any time. For more information, check out Visier's Privacy Statement.