Fact or Hype: Validating Predictive People Analytics and Machine Learning
Visier People uses predictive people analytics that are up to 17 times more accurate than guesswork at predicting risk of exit, and movement.
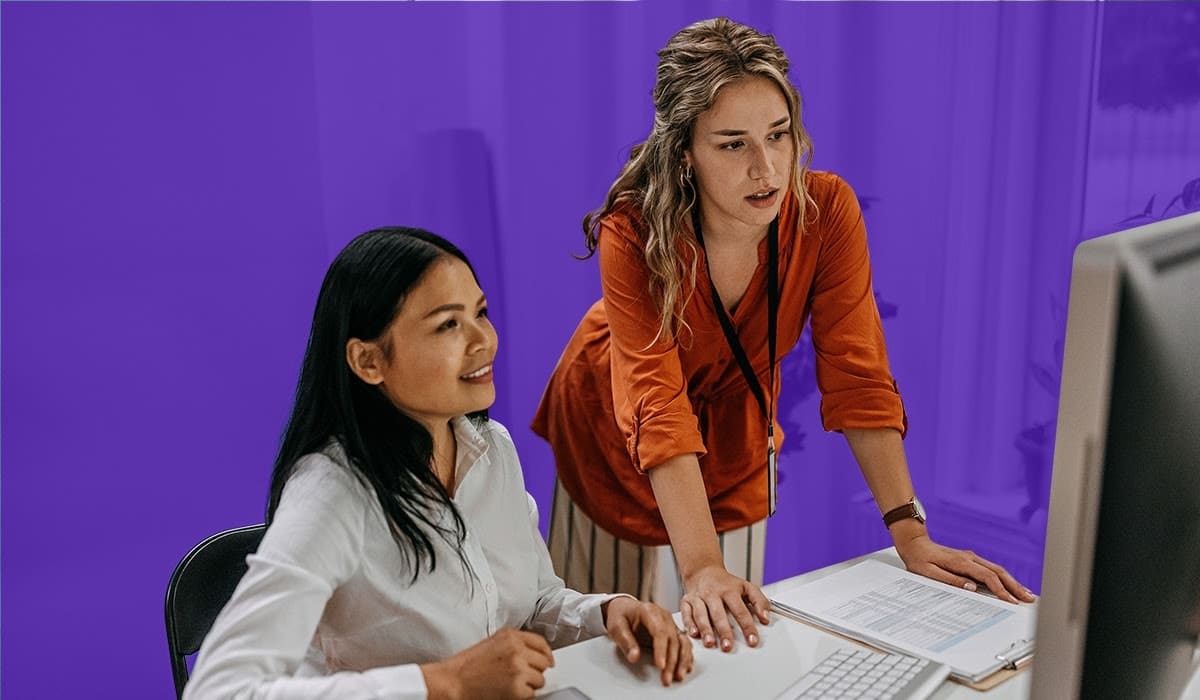
The 2016 Conference Board Survey of CEOs found that “Human Capital” is the CEOs number one global business challenge – for the fourth year in a row. For many HR professionals, this doesn’t come as a surprise.
Since the peak of the recession in 2009, the number of unemployed persons per job opening in the US has steadily declined to the point where there has never been a higher number of job openings. The war for talent is fierce and it’s become increasingly difficult for companies to both hire and retain talent.
Accurate predictions are the cornerstone of effective workforce analysis and planning. By forecasting how many employees are likely to leave, for instance, you’ll be better able to plan for the right number of new employees to hire. Expect too little attrition, and you’ll fall behind on hiring and workforce productivity will drop. Expect too much, and you’ll waste money ramping up talent acquisition programs.
In short, better predictions make it easier to match workforce supply with demand. Even a small increase in accuracy means significant savings, given that the workforce accounts for the biggest slice of the budget for most organizations.
If you can leverage predictive analytics to correctly identify employees who are at risk of leaving, should be considered for a promotion, or are likely to move laterally within the organization, you can avoid unnecessary and unexpected costs, while also enabling productivity and performance gains.
The keyword here though is correctly.
Why building predictive people analytics is challenging
First, with any predictive model, you need to have a means to verify that your predictions are valid.
When we put Visier’s Data Scientists to work on validating the success of our “at risk employee” predictive capabilities, they immediately identified that a minimum of 2-3 years of workforce data is required for the analysis to be valid (but the more the better).
It’s like the statement most parents have made to their kids at some point, “How do you know you don’t like it, if you haven’t tried it?” Or, in our case, how do you know the predictions are working, if you haven’t made a prediction that can be validated against real outcomes?
Second, the patterns behind why people make decisions aren’t always simple. People data is sensitive – it’s data with feelings.
It’s also messy, constantly changing, and housed in many disparate systems. To find the patterns inherent in such data requires looking across as many varied sources of information as possible. Like mining for gold, the wider your search is the more likely you are going to find the hidden nugget of insight.
Third, the accuracy of the predictions depends on the data used to create the model. A predictive model created based on the factors inherent at one company, doesn’t necessarily apply at a second company. Differences that arise over time can also compound the challenge — a model valid one year may not be valid the next, even within the same organization. Approaches need to take this into account.
Overall, the biggest challenge is that most predictive analytics capabilities available today are in their infancy. They simply have not been used for long enough or by enough companies or for enough employees or on enough sources of data.
How Visier’s predictive people analytics technology works
Our people strategy platform, Visier People®, uses predictive analytics technology that are up to 17 times more accurate than guesswork or intuition at predicting risk of exit, promotions, and internal movement.
To make our predictions even more accurate, our predictive engine uses a best-practice machine learning technique called random forest. Visier’s learning algorithm examines historical employee data and employee events like promotions, resignations, and internal hires to learn a set of patterns and construct decision trees that help you predict the occurrence of an event.
For example, the decision tree, in the following illustration, predicts whether an employee will resign in the next 12 months based on their attributes.
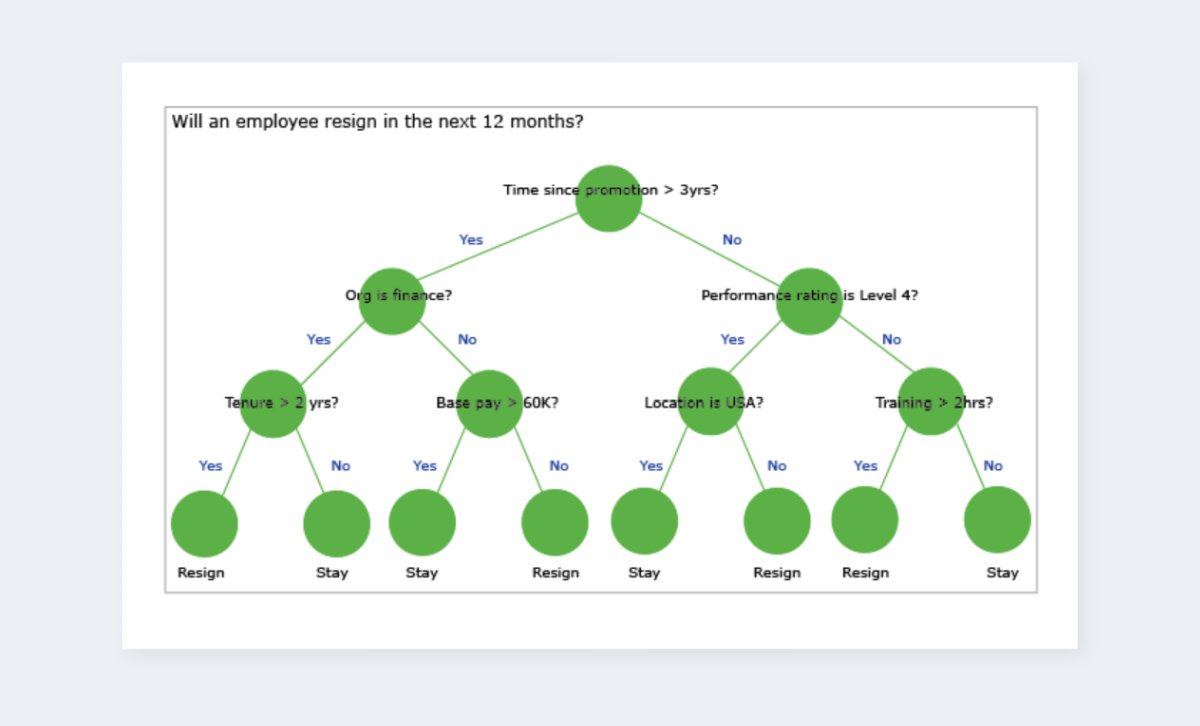
To construct a decision tree, the learning algorithm analyzes the employee data to determine the attribute that best separates the data into two distinct groups. For the previous example, the two distinct groups are the employees who resigned and the employees who stayed. This process is repeated at each node and the tree grows until the stopping criteria is met. The event likelihood is based on the proportion of employees in each group at the leaf node (the end of each path).
The random forest machine learning technique is based on the idea that an ensemble of decision trees is more accurate than any individual decision tree. Visier’s learning algorithm constructs many different decision trees by analyzing a random subset of information about the employee at each split to determine the attribute that best separates the data into two distinct groups.
This means that each tree is constructed using a different combination of attributes. Visier Workforce Intelligence looks at these attributes, determines the employee’s path for each tree, and calculates a prediction based on the historical event likelihoods across the trees. With Visier’s technology, you can also configure which employee attributes are most appropriate for your organization, and remove attributes that could lead to unintended bias—like ethnicity and gender.
Predictions in Visier People are calculated and re-calculated constantly, so when an HR analyst, business partner, or leader goes into the platform and asks which employees are going to change jobs in a specific employee sub-group (for instance, specifying a role, location, tenure, and performance level), Visier automatically provides the relevant results, based on the latest data.
Visier People does not limit or restrict what data is analyzed, but instead considers all the attributes for the group of employees being looked at. And because the platform was built to analyze all employee data, we are not limited like HR transactional systems that manage only a portion of the employee lifecycle.
These transactional systems cannot effectively answer strategic workforce questions, connect workforce decisions to business outcomes, or support future modeling and projections. Their underlying technology simply does not allow it in any meaningful way.
Visier People looks at all the employee attributes, collected in all HR transactional systems, from payroll to HR management to talent acquisition to recognition and so on.
Validate Visier’s results and build trust with your stakeholders
Each predictive model in Visier People is trained on all available employee data and employee events like promotions, resignations, and internal moves.
Predictive success is calculated by taking the predictions for employees at one instant in time and then measuring the actual event rate of these employees in the following validation period of one year. The predictive success measure is defined as the actual event rate of the employees with the highest predicted likelihood divided by the overall event rate in the organization.
Visier provides a validation metric for each predictive model that lets you measure how close the number of actual exits, promotions, and internal moves were to the predicted values inside the application. You can directly verify using the data of your organization alone and report on whether a higher prediction likelihood resulted in a higher rate of actual events.
This helps you increase the trust of your stakeholders by confirming exactly how accurate your past predictions were. With this kind of validation, you can be confident you’re giving leaders the right information to make their people decisions.
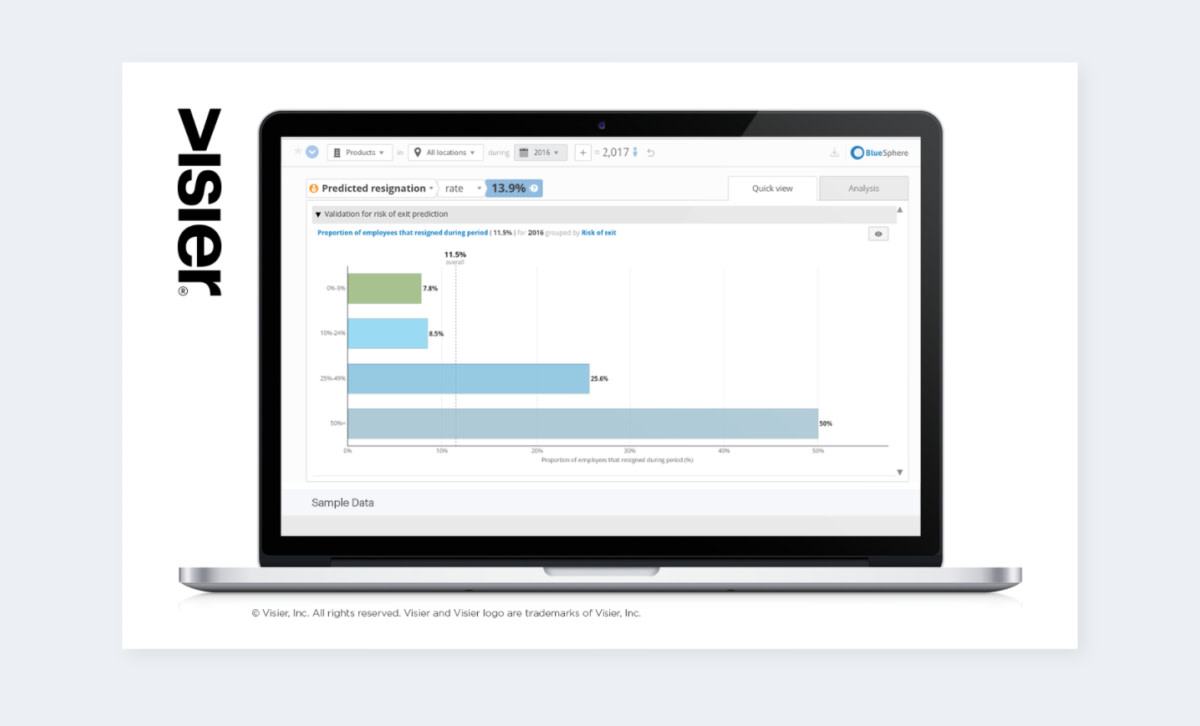
HR’s transition to predictive analytics
It’s important to remember that predictive analytics will not replace human intervention. Analytics can’t tell you the one clear course of action to take, but it gives you the deep insights needed to make the best possible decision based on facts.
Your own organizations journey to data-driven HR doesn’t (and probably shouldn’t) need to start with predictive analytics. Consider a “crawl-walk-run” approach in your graduation from metrics to true workforce intelligence, where you move from small success to bigger successes–whether it’s reducing your team’s time spent on producing custom reports or reducing turnover in a high-producing role.
When you do begin, ensure that your predictions are trusted and used by leadership because the approach you use is tried and tested, and validation can be shared with the organization.