Healthcare Employee Burnout Affects Everyone
Employee burnout affected nearly every industry during the pandemic. Find out why healthcare burnout is so high, and how it affects everyone.
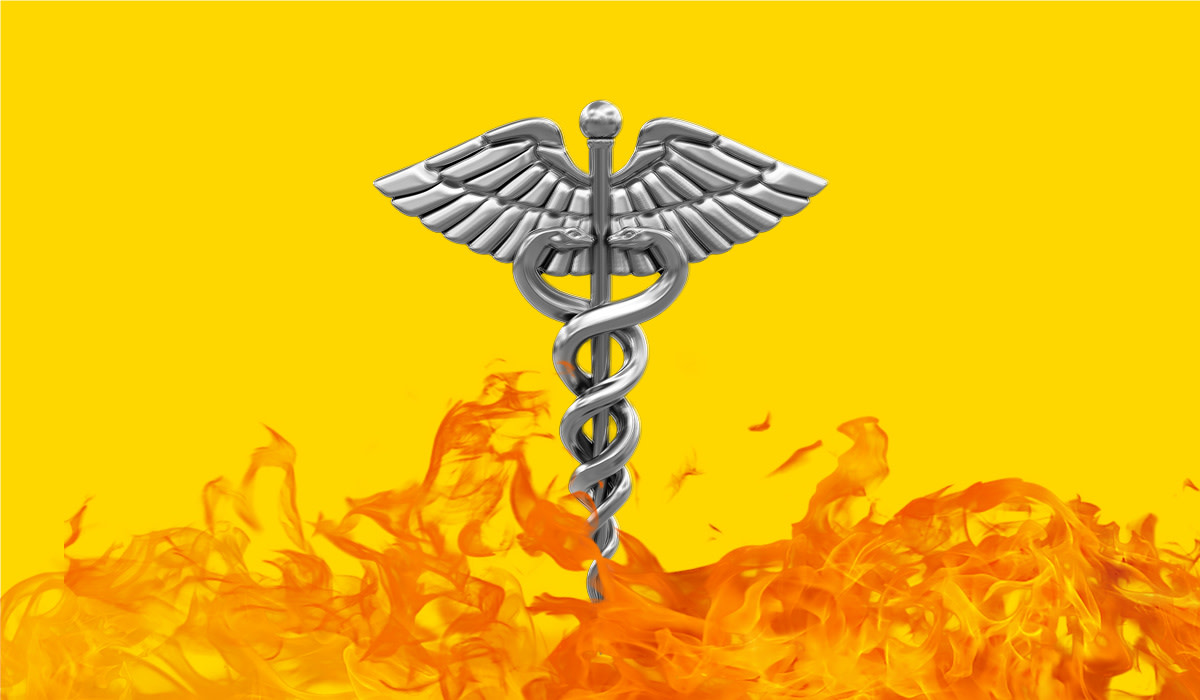
Resignations, quitting, medical leaves, and retiring from work due to employee burnout hit the healthcare industry especially hard. And now we are all seeing the lasting effects. Doctors are abandoning their patients. Patients are having to wait longer for their appointments for essential procedures. And we’re even seeing hospital closures, leaving entire communities without adequate healthcare facilities. According to new data published by the Association of American Medical Colleges (AAMC), the United States could see an estimated shortage of between 37,800 and 124,000 physicians by 2034, including shortfalls in both primary and specialty care. Why are so many medical staff retiring from work? Burnout.
What is employee burnout?
The World Health Organization (WHO) defines employee burnout as a syndrome resulting from chronic workplace stress that has not been successfully managed. It can take on many forms but typically it is characterized by feelings of energy depletion or exhaustion (doctors and nurses work long shifts and are often sleep deprived), increased mental distance from one’s job, or feelings of negativism or cynicism related to one’s job, and reduced professional efficacy. Even in a profession defined by a powerful code of conduct like healthcare, burnout can occur, though it may take longer for it to manifest itself. That’s why management needs to pay closer attention to the early warning signs of healthcare burnout.
Burnout exposes healthcare vulnerabilities
“The COVID-19 pandemic has highlighted many of the deepest disparities in health and access to healthcare services and exposed vulnerabilities in the healthcare system,” says AAMC President and CEO David J. Skorton, MD. “The pandemic also has underscored the vital role that physicians and other healthcare providers play in our nation’s healthcare infrastructure and the need to ensure we have enough physicians to meet America’s needs.”
The report highlights physician shortages in nearly every area of care including family medicine, general pediatrics, geriatric medicine, and medical specialties such as cardiology, oncology, infectious diseases, pulmonology anesthesiology, and neurology.
In May, United States Surgeon General, Dr. Vivek Murthy issued an advisory highlighting the need to address burnout among healthcare workers. According to Health and Human Services (HHS), the pandemic has made a bad situation even worse. Systemic challenges in the health system are leading to what the HHS calls “crisis levels of burnout.”
Burnout from first job to retirement from work
According to Visier’s The Burnout Epidemic report, it is clear that this trend of healthcare burnout is likely to continue. 89% of workers report having experienced burnout since 2020 and 7/10 employees say they’d quit due to burn out. Frontline healthcare workers had it worse than most.
According to a report, based on findings from the Kaiser Family Foundation/Washington Post Frontline Health Care Workers Survey, taken every year since 1995, of 1,327 frontline healthcare workers (defined as those who had direct contact with patients and their bodily fluids) and representing those working in hospitals, doctors’ offices, outpatient clinics, nursing homes assisted care, and home health care), the majority have experienced adverse mental health impacts as a result of the pandemic
Employee burnout signs
62% report high levels of stress
56% say they are having trouble with sleeping
47% are sleeping too much (47%)
31% are experiencing frequent headaches or stomachaches
16% have increased their use of drugs or alcohol to cope
13% say they have received mental health services or medication specifically to address worry or stress related to COVID-19.
18% say they expect to need those services in the future.
It’s even harder for younger healthcare workers, many of whom got their first jobs out of medical or nursing school during the pandemic. KFF/Washington Post reports 3/4 of healthcare workers age 18-29 years old say they are “burned out”. Caring for people with a deadly disease like COVID-19 is stressful enough of course, but the situation becomes more acute when workers are ill equipped. A lack of personal protective equipment (PPE), vaccines and other medications has made things worse. For many, the stress has become unbearable, as indicated by a recent study by Incredible Health that says 34% of nurses plan to leave their current role by the end of 2022. Of these, 44% cited burnout and a high-stress environment as the reason. It seems clear these nurses are resigning because of burnout.
Effects of COVID-19 on nurse burnout
Frontline workers are the true heroes of the pandemic and they need to be both celebrated and supported. “The nation’s health depends on the well-being of our health workforce. Confronting the long-standing drivers of burnout among our health workers must be a top national priority,” says Murthy. “COVID-19 has been a uniquely traumatic experience for the health workforce and for their families, pushing them past their breaking point. Now, we owe them a debt of gratitude and action. And if we fail to act, we will place our nation’s health at risk.”
The COVID-19 pandemic has played an outsized role in these resignations. Despite the fact that 89% of nurses surveyed by Incredible Health have received one or more doses of a vaccine and over half (54%) reported never contracting the virus while at work, those that did contact the virus were often expected to continue working or were expected to use vacation days and/or sick days to stay home (38%). Being a healthcare worker in a time of crisis is like being a soldier during wartime. This isn’t burnout, it’s betrayal.
Burnout is systemic
Managers, mistakenly thinking that burnout is something that only affects individuals, have tended to treat the symptoms, not the causes. Dr. Antonella Aguilera-Ruiz, Chief Health Officer at peace of mind, a program that helps employees develop the skills and lifestyle necessary to manage stress and curb burnout, says employers must address the root causes of burnout, both individual and systematic, in order to effect change.
Like a virus, burnout can spread throughout your organization. Just before the pandemic started, the Harvard Business Review looked at how workplace stress can impact mortality and health costs in the United States and found that it led to nearly 120,000 deaths and nearly $190 billion in spending each year. Preventing nurse burnout—and healthcare burnout—will have a stronger impact than addressing long-term stress. While yoga, meditation, and breathing exercises can help an individual, they do little to combat the overall well-being of your organization.
Management’s role in preventing employee burnout
A Gallup survey of 7,500 full-time employees found that the top five reasons for burnout are all things that management can address before burnout actually sets in:
Unfair treatment at work
Unmanageable workload
Lack of role clarity
Lack of communication and support from their manager
Unreasonable time pressure
While this survey was taken before the stresses of the pandemic, they have all become worse. Add to that the helplessness many healthcare workers feel when dealing with a highly transmissible and deadly disease on a daily basis, and you begin to understand why burnout hit the healthcare industry especially hard. The Surgeon General’s Advisory Addressing Health Worker Burnout provides several recommendations for how healthcare organizations, insurers, policymakers, academic institutions, and others can help address healthcare burnout through systemic changes in work culture, policies, and protection.
Listening to employees is key to addressing employee burnout
It sounds obvious but healthcare institutions, like other companies, must start by listening to their workers. Workers must weigh in when improving processes, workflow and culture. And, employers should address several factors specifically affecting healthcare employees:
Substance abuse
Right now many healthcare organizations actually impose punitive policies that make it difficult for alcohol and drug users to come clean. That needs to end. Employers should provide mental health and substance use disorder care.
Personal protective equipment
A lack of proper PPE is no longer the problem that it was at the beginning of the pandemic but managers still need to ensure that they are providing a clean, safe, and comfortable environment for their workers.
Workplace violence
In a survey prepared by National Nurses United in mid-2021, Workplace Violence and COVID-19 in healthcare, eight out of 10 healthcare workers said they had experienced at least one type of workplace violence during the pandemic. Two-thirds had been verbally threatened, and one-third of nurses reporting an increase in violence compared to the previous year. Among 26,174 state, tribal, local, and territorial public health workers surveyed, nearly a quarter (23.4%) reported feeling bullied, threatened, or harassed at work.
Healers must remain healthy themselves before they can heal others. A healthy work environment requires living wages, paid sick and family leave, plenty of rest breaks, and regular scrutiny of their workloads and working hours at minimum. Family-friendly policies such as childcare can help. Adequate staffing is critical to preventing healthcare burnout.
The support healthcare workers need
With the move to tele-medicine accelerated during the pandemic, many healthcare workers, especially doctors, have found that they are missing the social interaction that they had with their patients and colleagues. Healthcare managers should prioritize community as a core value of the healthcare system.
The last few years have been particularly hard on healthcare workers. Instead of treating burned out employees as a problem, managers need more empathy. Do whatever it takes to address staffing issues, caseloads, and provide a supportive work environment. Even now, as the worst of the pandemic seems to be behind us, understand that healthcare workers may be suffering from a kind of post traumatic stress after seeing so many of their patients (and even their own loved ones) suffer and, in all too many cases, die. Healthcare organizations should start now with the things they can address so they will be better prepared when the next wave washes over them.
People analytics can help employers identify and address burnout, as well as other critical employee issues. Interested in learning more about Visier? Get in touch with us for a demo!
On the Outsmart blog, we write about workforce-related topics like what makes a good manager, how to reduce employee turnover, and employee burnout. We also report on trending topics like the Great Resignation and preparing for a recession, and advise on HR best practices like how to present headcount data to your CEO, metrics every CHRO should track, and connecting people data to business data. But if you really want to know the bread and butter of Visier, read our post about the benefits of people analytics.