HR as a “Prediction Machine”
The impact of AI will likely hinge on dilemmas that are not widely recognized today. Dr. John Boudreau shares how HR leaders can articulate and solve them.
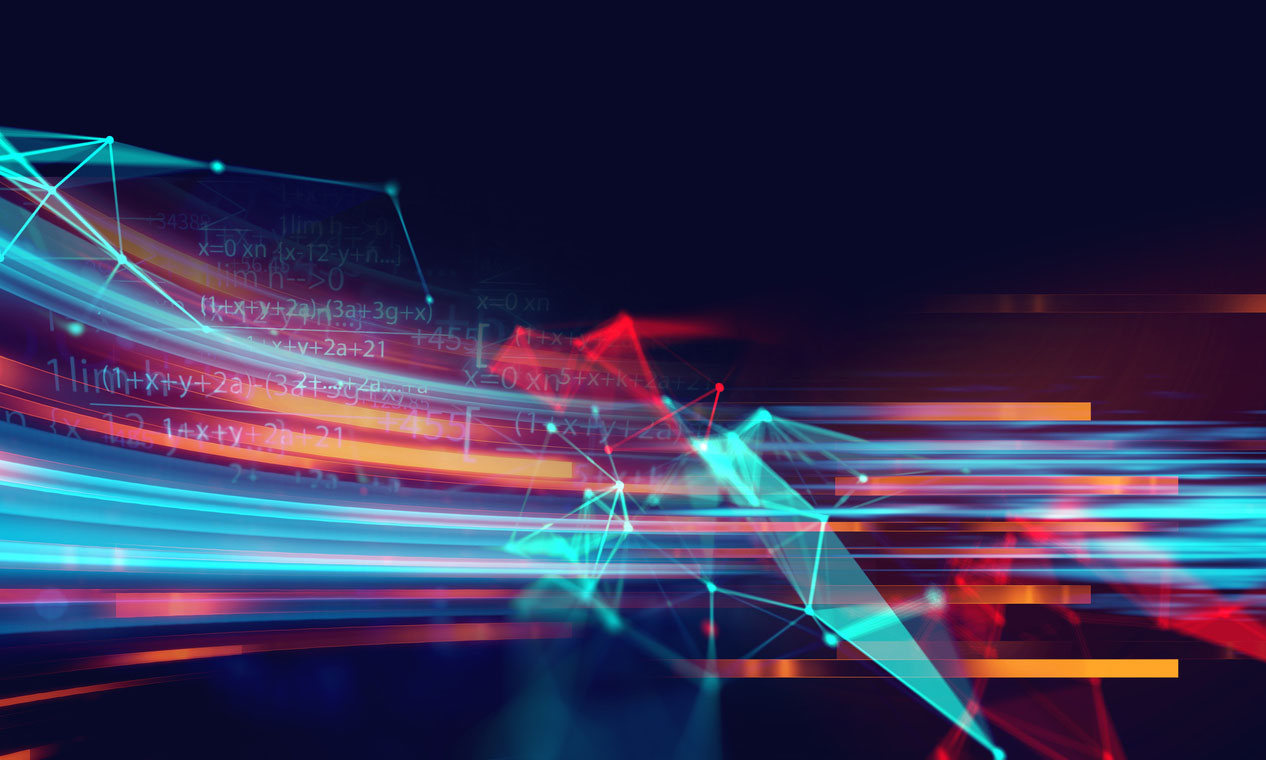
A web search on “AI in HR” reveals a mind-boggling array of possibilities.
For example, AI can choose candidate sources, predict candidate performance, offer coaching and learning, and even suggest employee pay levels. Yet, a recent IBM survey suggests that only 66% of CEOs believe cognitive computing can drive significant value in HR, and only 50% of HR executives recognize that cognitive computing has the power to transform key dimensions of HR.
Are these leaders missing the point? Or, are they reserving judgment, because the reality of Artificial Intelligence (AI) in HR will be more complex?
In fact, the impact of AI will likely hinge on dilemmas that are not widely recognized today. One of those questions is whether AI is built to predict an outcome, or to mimic human behavior. Such dilemmas apply to AI in human resources, but they are vital to all AI applications. HR leaders should prepare to play a significant role in articulating and solving these dilemmas.
Functional leaders such as CIO’s, CTOs, COOs, and CFOs often drive the AI application debate today, but HR can add important perspectives that go beyond simply technical or economic considerations. HR has a golden opportunity to make a strategic contribution by articulating these hidden dilemmas and providing needed frameworks to solve them.
HR leaders can prepare for this leadership role today as they apply AI in HR.
When AI Makes “Prediction” Cheaper, More Prediction is Used
In the book, “Prediction Machines,” economists Ajay Agarwal, Joshua Gans, and Avi Goldfarb remind us of an important principle: If something is less expensive, then people use more of it, and in new ways.
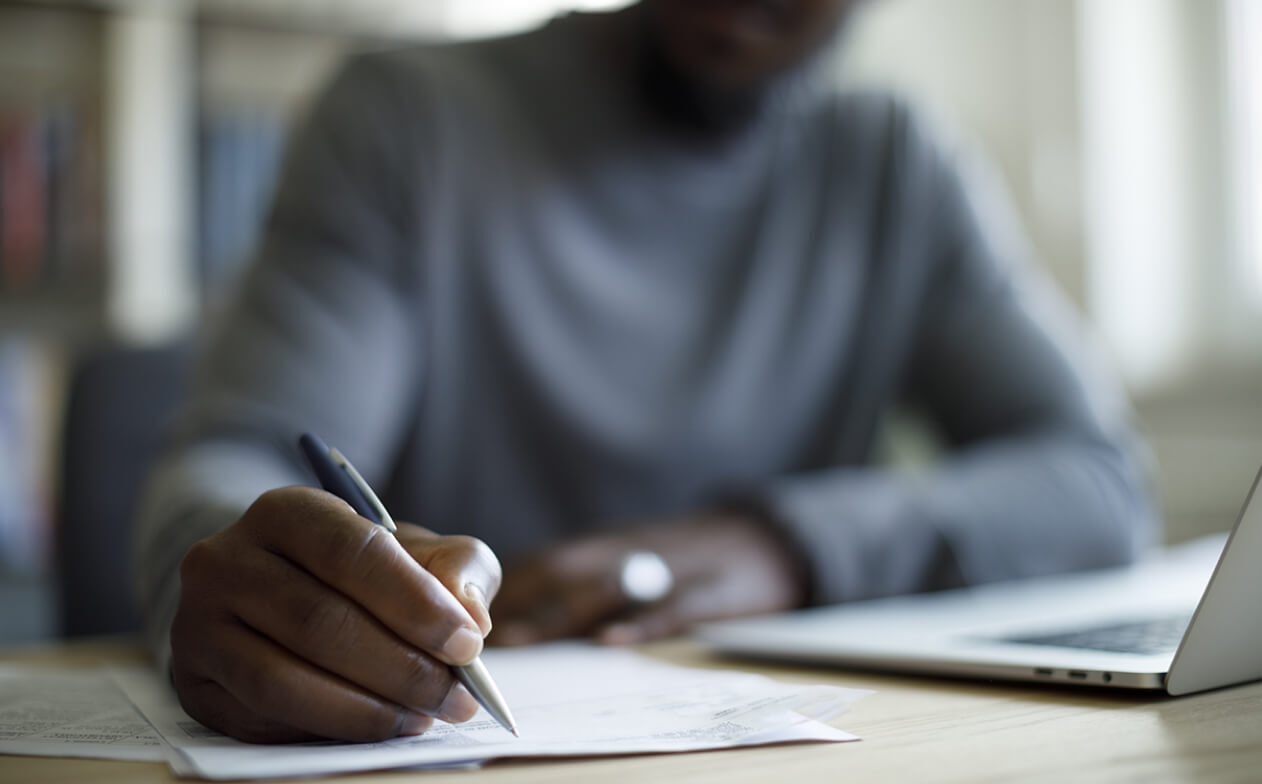
They note that high-speed computing in the 1980s and 1990s made “math” cheaper. Before then, few thought of movies and music as “math,” but then high-speed computing made “math” so inexpensive that it became cost-effective to translate movies and music into digital “math,” transforming how we purchase, share, and enjoy them. The authors note that AI is similarly making “prediction” cheaper, and that prediction will be used more, and in new ways. Music, movies, art, facial recognition, and many HR decisions can be translated into “prediction.”
The authors also recognize a related principle: AI applications will favor the cheapest way to train the AI, and therein lies an important dilemma for HR and other leaders.
Consider self-driving vehicles. Such vehicles have existed for decades, and they are widely used in very controlled environments, such as a factory floor. They work in that environment because the required predictions are finite and simple. The vehicles follow only a prescribed route, picking up and moving items from one set of specific locations to others. The predictions are simple things like:
If the assigned item number matches the number on the item, then it is the correct item to pick up;
If the assigned number matches the number on the shelf, then it is the correct shelf to place the item;
If the sensor detects any movement ahead, then it is a person or equipment, so stopping will avoid a collision.
Notice that the predictions are not perfect. It’s possible that movement ahead of the vehicle does not require stopping, but they are good enough to make the predictions cost effective.
What happens when self-driving vehicles are deployed on actual roadways? They encounter massively more vehicles, humans, weather conditions, etc., that produce a virtually infinite number of necessary combinations and prediction rules.
If the goal is to train AI with the decision rules necessary for good driving, that’s impossible. Also, what is “good driving”? If the goal is to maximize safety, then the vehicle should stop when encountering a pedestrian or other vehicle. Of course, that will increase journey time, and cause abrupt braking that might increase accidents. If the goal is to minimize travel time, the AI may choose uncrowded routes that save a few seconds but increase risk, because driving through residential neighborhoods creates more encounters with pedestrians.
AI is applied to HR and work. For example, if AI is used to select among job candidates, important objectives might be future job performance, tenure, career progress, diversity, or legal compliance. These goals are not mutually exclusive, but they often require tradeoffs. The number and variety of factors that affect these outcomes are huge. So, constructing AI to make “good hiring decisions,” is a daunting task.
Does that mean that AI cannot learn to drive or select job applicants? No. There’s a simpler solution, but it poses fundamental dilemmas.
It’s Often Easier for AI to Mimic Humans
A less expensive and simpler alternative is often to “train” the AI to drive like humans. Machine learning and other methods can feed AI millions of data points that describe the decisions of actual drivers, combined with sensor-based real-time information about the driving conditions, surrounding vehicles, and traffic signals. Just as AI learned to play the game Go, by analyzing games played by humans, AI can learn to “drive” by analyzing millions of human driving decisions. It is often far easier and cheaper to produce AI that mimics humans than to specify an objective and devise all the if-then rules necessary to achieve it.
When it comes to selecting job applicants, it may be faster and cheaper for AI to mimic human hiring managers, rather than specify what is a “good hire” and the if-then rules needed to achieve “good” hires.
Of course, this fast and less expensive alternative comes with tradeoffs. The humans the AI mimics may be flawed or biased. AI that drives like humans might “learn” to speed up at yellow lights. AI that selects like human hiring managers might “learn” biases like “only some nationalities are good at math.”
What’s better: articulating the objective and its decision rules or mimicking humans? The answer will vary, and the causes are nuanced. Research is only beginning, but some results are tantalizing.
One study of arrests in New York City between 2008 and 2013 compared the results of judges versus algorithms in deciding whether accused criminals should be released on bail or held in jail, pending trial, using the outcome of whether those released would fail to appear for trial. An algorithm trained with the actual outcomes could achieve the same risk with significantly reduced jailing rates, or reduced risk with similar jailing rates, and with less racial bias. An algorithm trained to mimic the judges’ decisions improved on actual judges’ decisions, but not as much as the algorithm trained using the actual outcomes.
AI in HR: HR’s Opportunity to Lead
This fundamental decision–articulate the objective and its causes versus mimic the humans–is often invisible, embedded deep within algorithms, unseen and unconsidered by the leaders that create, purchase, and use the AI. These are questions of values and human behavior, not just economics and technological capability. These questions require the benefit of a profession that can deal with values, human behavior, and biases. That profession can be HR–if HR leaders can articulate this fundamental AI dilemma and guide leaders to recognize and deal with it.
HR should prepare for leadership in articulating these questions, and developing frameworks to help answer them. There will be no perfect answers. Professions like information technology, operations, finance, and computer science have much to offer, but these thorny questions require more. HR’s disciplinary foundations in human behavior, culture, and ethics offer a unique and essential contribution.
How can HR prepare to lead? Applying AI to HR itself is the opportunity to learn and lead, by tackling the tough questions. AI in HR is already choosing recruiting sources, selecting among job applicants, allocating incentive pay among workers, choosing learning and coaching offerings, and the list expands. As prediction gets cheaper, more of “work” and HR will offer cost-effective prediction applications.
Each of these applications poses the same dilemma–choose between the laborious task of specifying “good” outcomes and decision rules in advance, or mimic humans. The latter will often be faster and cheaper and tempting, but may obscure important values, tradeoffs, and biases.
HR leaders have a golden opportunity to understand and articulate these tradeoffs as they apply AI to HR, but this is a much larger issue than just AI in HR. The insight and experience gained in exploring these questions within HR can prove valuable when this function is called on to articulate and help address them outside the HR profession.
HR should be prepared to help guide those decisions wherever technology is applied.
Get the Outsmart newsletter
You can unsubscribe at any time. For more information, check out Visier's Privacy Statement.