ARTIFICIAL INTELLIGENCE
What Is AI Analytics?
Extracting meaningful insights from endless pools of data can be cumbersome. AI analytics simplifies this process, analyzing large amounts of data effortlessly and helping you make the most of your data.
Get a Demo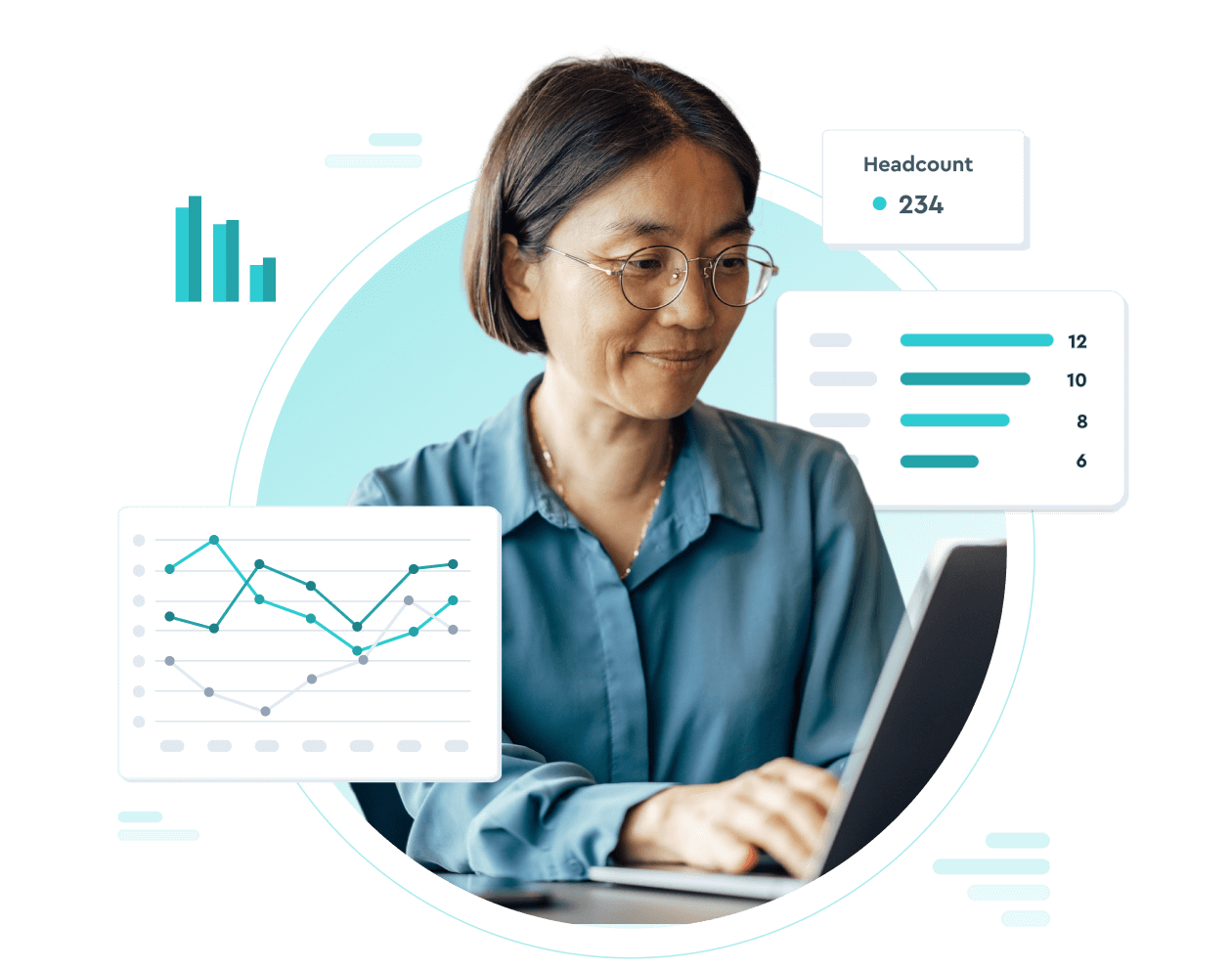
Table of contents
What is AI analytics?Benefits of AI analyticsHow to use AI in data analytics Use cases for AI analyticsUsing AI and people analyticsWhat is AI analytics?
AI analytics uses techniques like machine learning and natural language processing (NLP) to automate processes and analyze large amounts of data, resulting in valuable predictions and insights that lead to better business outcomes.
It leverages the power of algorithms and AI's high data processing capacity to give you faster and often more accurate results. Manual data analytics may reach the same results, but its data processing capacities are limited.
Key elements of AI analytics include:
Data sources. Where are you getting your data from?
Data preparation and collection. How will you gather the data and do you need to do anything before you can analyze it?
Data analysis. This analysis can include machine learning, NLP, charts, and other visualization tools.
Insights and decisions. What are the conclusions, the patterns you observed, and the next steps based on the analysis?
Despite the benefits, AI analytics is not without fault. It can make mistakes and its interpretations of data can be wrong. Unlike humans, an AI algorithm won’t have a sense of right or wrong, making decisions based on a fixed set of information. That’s why AI analytics still requires human intervention before any final decisions can be reached.
What’s the difference between AI analytics and traditional analytics?
Analytics, in general, refers to the process of identifying, interpreting, and communicating patterns and results regarding the data at hand. Traditional analytics realizes all that with little automation.
There are four main types of analytics:
Descriptive analytics: You seek to understand what happened.
Diagnostic analytics: You try to find the cause of an event.
Predictive analytics: Help you gain insights into possible future events.
Prescriptive analytics: Used to understand what your next steps should be to reach a certain goal.
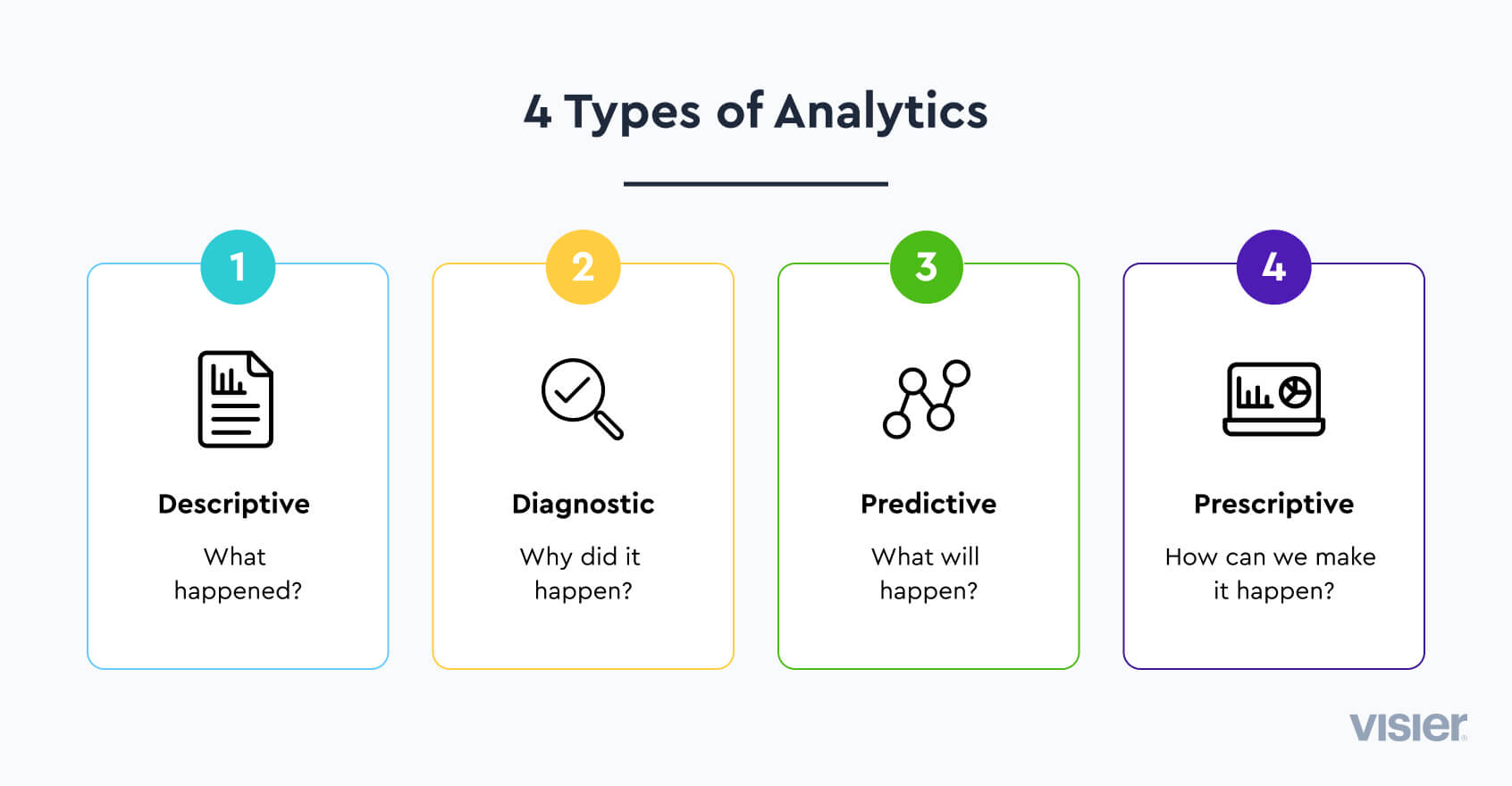
AI analytics can help with all four, though it is more commonly used for predictive and prescriptive analytics.
Data sources, collection, preparations, analysis, and insights are steps traditional analytics and AI analytics share. There are two key differences: the methods used for data analysis and the amount of data handled.
With traditional analytics, analysts will have to look at data, form hypotheses, test their ideas, refine them based on results, and, eventually, find patterns and draw conclusions. For many businesses, this process is not constant and rarely looks at all the data related to the company.
AI analytics, on the other hand, removes these limitations and can constantly monitor and analyze large amounts of data.
Benefits of AI analytics
AI analytics can quickly analyze and identify patterns even when using very large sets of data. Here are some of its benefits.
Risk management
AI analytics helps you find and mitigate risks in a wide variety of sectors, including finance, cybersecurity, business, and more. It helps you stay ahead of threats, comply with regulations, and create smarter risk management strategies.
Anomaly detection
AI can identify anomalies quicker than traditional analytics methods can. You can detect things like fraud attempts or any unusual behavior in real time. This, in turn, improves the security of your system and increases customer trust.
Increased productivity
AI analytics won’t replace your data analysts altogether, but it will help them be more productive and gain more insights. The speed with which AI can process data means you’ll get more results quicker, which will help you make better decisions and take action sooner.
Improved customer experience
Using AI analytics, you can gain deeper insights into what your customers want. This will lead to improved experience, marketing success, and customer retention.
How to use AI in data analytics
To use AI in data analytics, you need to start with a goal and prepare your data and algorithms. The exact process might differ slightly from company to company, but there are some steps you’ll need to include regardless of your sector.
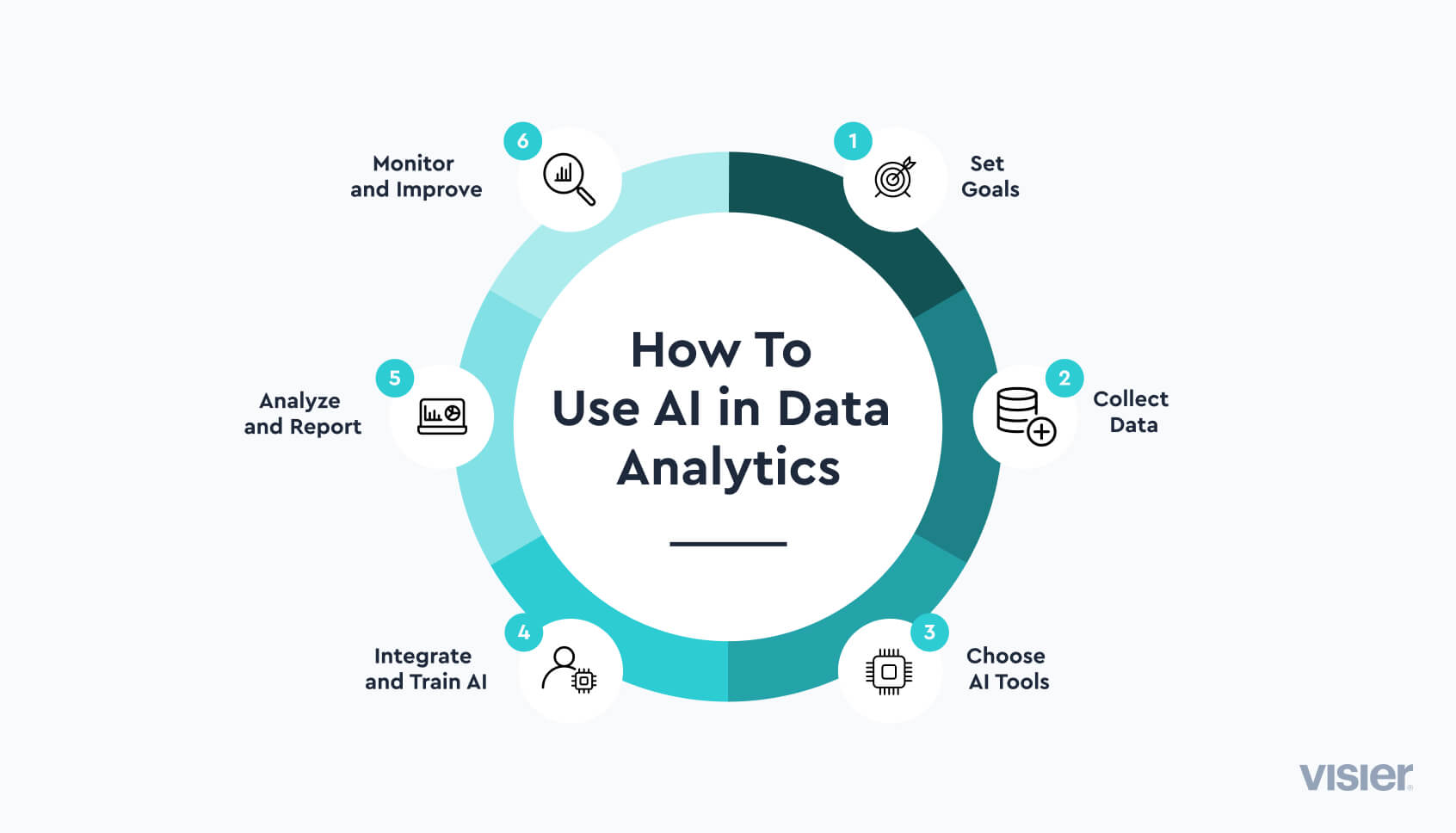
1. Set your goals
The first thing you’ll need to do is answer one simple question—why do you want to use AI analytics? The answer can include both the sector, but also smaller goals. For instance, if you’re using it in HR, you may want to improve your talent acquisition process. You can also look at certain metrics like time to fill or time to productivity to have a more exact number to work towards.
2. Data collection
You can’t have analytics without data, so selecting your data sources will be the next step. Make sure they’re accurate, unbiased, and up-to-date to have the best results. Collect the data while making sure you’re compliant with local laws such as the General Data Protection Regulation (GDPR) or the California Consumer Privacy Act (CCPA).
3. Select AI tools and techniques
Selecting the right tools is a critical step. Your goals, the amount of data, and your business sector can help guide you toward the right decision. Employees’ skills are another factor. You will need some human intervention, so you’ll want to bring in people who can use the tools you select effectively.
The techniques will also vary depending on your data. In some sectors, machine learning will be a must, while others may prefer NLP or a combination of several techniques.
4. Data analysis and integration with business process
Once you have your goals, your data, and your tools, you can begin the analysis process. For this, you’ll first need to train the AI models on historical data before they can make accurate predictions or generate insights.
This is usually a long-term process you’ll need to repeat several times, depending on your goals. You need to integrate AI analytics with your business processes and systems to make it a part of your daily operations.
5. Visualization and reporting
For this step, you can use charts, graphs, reports, and any other tools that will help you present your findings and use them to improve your business.
6. Monitor and improve
Data analytics is something you’ll use again and again. That’s why you’ll need to monitor performance and make improvements wherever necessary. Measure ROI, look at metrics, and gather feedback from stakeholders to understand the impact AI analytics is making in the company.
Use cases for AI analytics
AI analytics can be applied across a wide variety of sectors and serve many purposes. Here are some of the most common use cases.
1. Talent acquisition
A critical step for any business, talent acquisition can be a long and costly process. AI analytics can make it easier and faster.
The most common (and controversial) way to use it is to analyze resumes to identify the best candidates for a position. This is useful when you have a lot of applications and want to identify the top ones quickly.
Many argue this is not only great for speed but also eliminates biases a human may have when they first look at someone’s resume. Plus, AI will not get tired, so a good application won’t slip through the cracks. Others argue you could miss a truly great candidate if they did not include a certain keyword in their application, even if they check all other boxes. Weighing the pros and cons of using AI analytics in talent acquisition is recommended, along with periodic human interventions to ensure that your tools and processes are operating as planned.
You can also use AI analytics to predict which candidates are more likely to succeed after the onboarding period is over or analyze why, for some, the time to productivity is shorter than for others. This will help you optimize the onboarding phase, creating a more effective talent acquisition program.
2. Employee engagement
Engaged employees perform better and are less likely to quit unexpectedly. Increasing engagement is not always the easiest process. Things like learning and development programs or succession planning may help, but sometimes you need to do more.
With AI analytics, you can look at data related to employee engagement, including their feedback, performance, absenteeism, and more. Analyze their skills and create a personalized development plan to aid in their career objectives.
You can also use AI analytics to monitor performance and productivity and offer personalized merit rewards. All this will boost employee satisfaction, engagement, and retention.
3. Campaign segmentation
Your human resources department isn’t the only one that can benefit from AI analytics. It can also analyze your customer’s preferences and help you create targeted, personalized campaigns.
4. Fintech analytics
The financial sector is constantly exposed to threats and risks that can affect not only customers but the entire business. Through prescriptive AI analytics, you can monitor the behavior of your systems, noticing any abnormalities as soon as they happen. This helps you mitigate risks and avoid threats before they become a security breach.
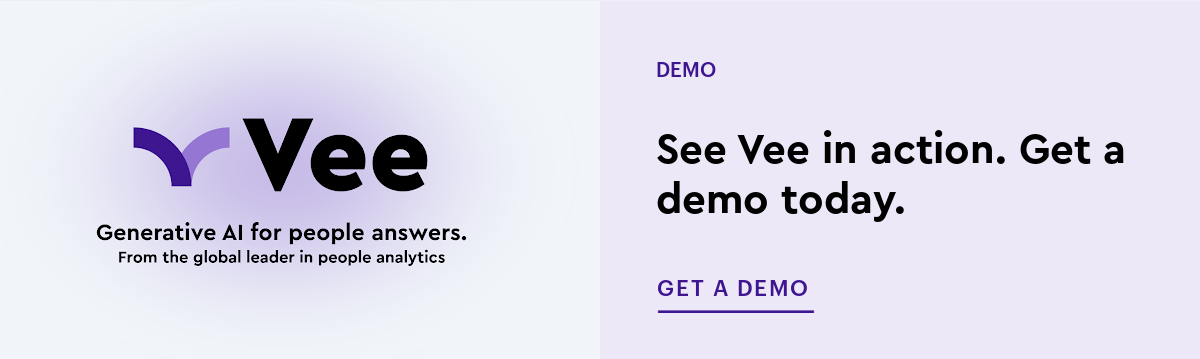
Using AI and people analytics
AI analytics can improve many areas of your business. Regardless of where and how you want to use it, your first step should always be to look at the people in your company. People analytics is an effective tool when you want to take the people data you have and turn it into valuable insights. You’ll gain a deeper understanding of your workforce and you’ll make data-driven decisions in every area of your business.
To take the power of people analytics one step further, Visier created Vee, an AI digital assistant that helps gain more meaningful insights and interpret data correctly. Vee simplifies people analytics with a conversational interface that complements charts and graphs.
Once you have the data that matters, you can start focusing on other pressing issues and use the power of AI to dig deeper. One of the critical aspects of any business is talent retention.
Use data to understand what drives your employees, what motivates them to always do better, and what will make them stay with the company. AI analytics can take this process further by predicting flight risk, identifying engagement drivers, and creating personalized career plans.