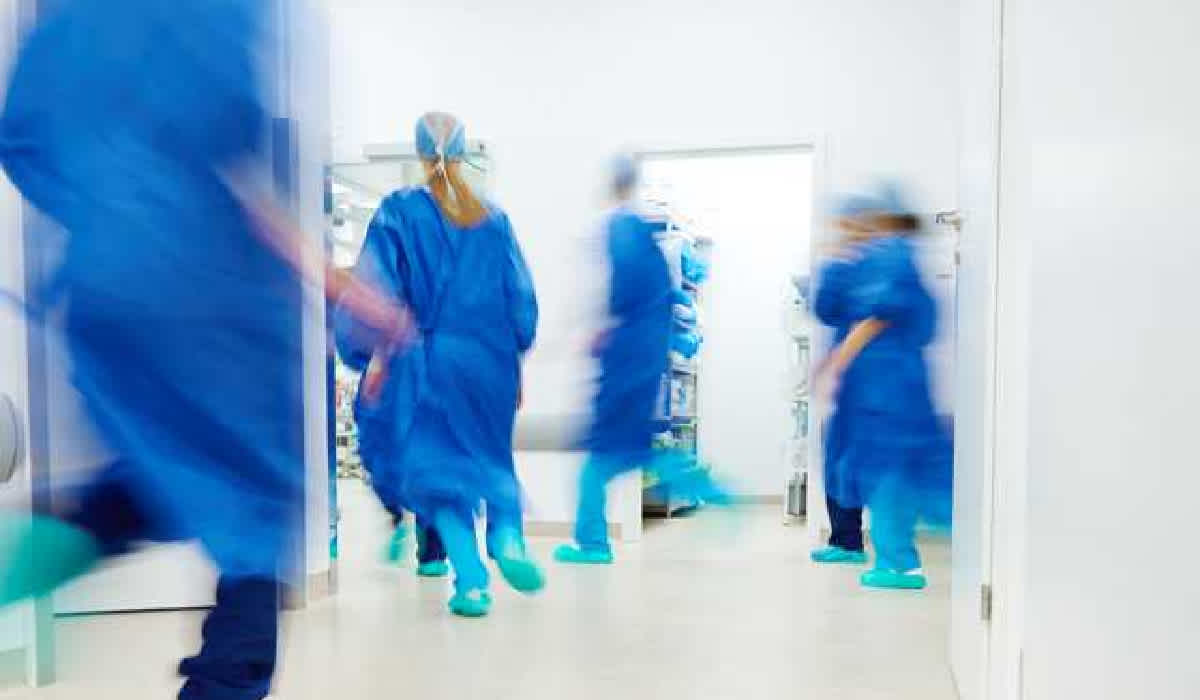
Customer story
Healthcare
Providence
Providence operates more than 50 hospitals and 800 clinics in the western U.S.
Summary
Industry: Healthcare Headquarters: Renton, WA Employees: 120,000 Challenge: Determine if and how raising salaries will affect turnover, and what it would cost Solution: Leverage historical data and survival analysis
Visier Solutions
Industry: Healthcare
Headquarters: Renton, WA
Employees: 120,000
Challenge: Determine if and how raising salaries will affect turnover, and what it would cost
Solution: Leverage historical data and survival analysis
Overview
In 2020, Gary Russo, the executive director of Workforce Intelligence at Providence, conducted a study to test whether raising pay could actually save his organization money. Gary explained that this counterintuitive idea hinges on the costs of attrition. Most people would anecdotally agree that higher pay reduces attrition. “Pay is one of the reasons people leave,” he confirms. “We’ve seen pay go up and down in importance, and right now pay as a reason [for staying or quitting] is on an upswing.”
Seems simple: Pay your staff more and they want to stay working for you. Right? But it’s not so easy to find the sweet spot, says Gary. There is a point at which modulating pay simply drives the organization out of business, and that can be influenced by how pay adjustments are handled. For instance, spreading around equal-sized pay bumps to everyone (aka the “peanut butter spread,” he quips) gets complicated with various compensation structures. A five-percent pay increase buys a very different cup of coffee if you make $20,000/year versus $200,000/year.
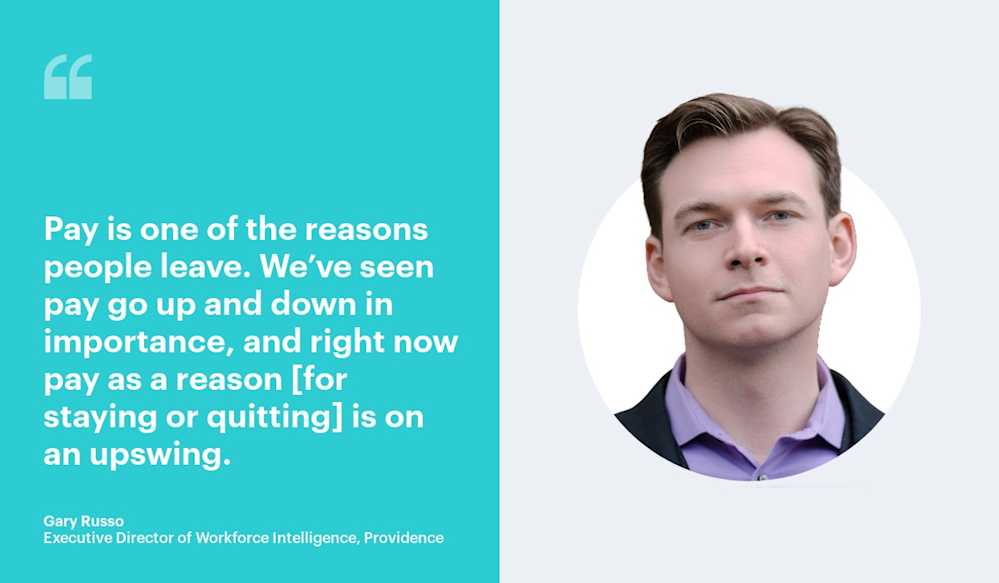
Moving away from an equal spread and instead giving a raise to just the lowest-paid workers could start a domino effect—once they’re paid more, they’re no longer the lowest paid, so the next group would be considered, and so on. Stepping away from the math of pay raises, for a lot of people their reason to leave is not primarily dependent on pay. If you look at where these groups are are on the compensation curve and watch them over time, a small raise isn’t enough to convince them to stay. There is a point of diminishing returns where increasing pay simply stops being a good lever for retention. Gary turned to people analytics to find the sweet spot where raising pay yields a positive return on investment—either in net retention or even net cost.
Failing fast toward the slow burn of success
Gary’s background in academic science taught him not to target the whole population. He set about finding pockets within the organization where there was so much sensitivity to pay with regards to attrition that raising pay was a cost-effective driver. “For the pay-to-stay analysis, Visier helped us fail fast,” he says. Because Gary and his team had loaded a lot of their information in Viser already, they could look across different pay bands and look at turnover rates. That let him assess what to investigate further.
“I could add a filter and check some areas we know, for other reasons, seem pay-driven. For example, a thousand-dollar pay boost can be a life-changing amount for roles paying near minimum wage. Could we try to pick out different parts of our organization and see different sensitivity to pay? We quickly found enough compelling results to justify a deeper investigation.”
Using historical data to predict the future
They assessed data on the relation between salary range and the associated turnover range. The Compa-ratio let them bucket people into groups paid either above the midpoint or below the midpoint. Instead of worrying about the exact dollar amount, it was more about if they were paid in relation to the job they were in, and the pay grade midpoint was a useful proxy for a market competitive wage.
Once they had these pay buckets, they took the experimental group for a given time bracket, say from 2018-2020, lined them up by date of hire, and saw how many stuck around over time. Since they already knew who had left and who had stayed, what their pay had been with regards to the midpoint, and had the data to control for other variables, they could visually demonstrate to the leadership the real cost of paying people below the midpoint range for their role.
The result was that Gary’s team was able to identify a group of employees where the organization would at least break even by paying them more to stay and, in some cases, would even save money. There’s a type of regression analysis called the Cox proportional-hazards model, a type of survival analysis. This type of analytics is often used in medical testing because the concept of survival analysis refers to whether or not a patient survives with or without a treatment. It essentially asks, “Does our treatment improve or worsen the outcome over time?”
They started with a cohort of employees and considered the first day of hire as the beginning of the “treatment,” e.g. when they began receiving pay. On day one the cohort is at 100% and then over time people slowly turn over, which shows up on the graph as a curve. “Eventually, let’s say after two years, you’ve got 60% of people left,” explains Gary, using 60% as an example. “That’s essentially your retention curve. Once we saw the most prominent trends, it was easy for us to look back at the data (in Visier) and see the difference in turnover between these pay buckets. The benefit of going through the extra analytics hassle is that you can start running better statistics on how likely these trends were for new hires.” It was clear that, through analytics, Gary was able to identify critical areas where reevaluating pay would have the largest, positive effect.
Gary explains that many of the discovered areas were already top-of-mind for leadership and the total rewards team. “Some of the reason this happens is that the market is moving faster than our pay structure. Rumors that the competitor down the street is paying more spread quickly, so often HR is actually last to know. This analysis served as a new line of evidence that we needed to address pay,” Gary says. When he brought that back to the compensation team, they weren’t surprised. They’d suspected as much and this analysis provided a new layer of data to show the business impact of prioritizing solutions.
Survival analysis proves the value of “Pay to Stay”
Every time a company hires a new employee, it is because they recognize value. Employees bring value to the organization. In many cases, the value of the work that an employee provides is two or three times the wages they receive. Some roles, such as the case of a highly skilled specialty nurse, the value of work can balloon past 800-percent of their salary. For his “Pay to Stay” study, Gary used a cost-of-turnover calculation he created to help Providence better understand its attrition-related costs. He said it generally agreed with Becker’s Hospital Review’s estimate of each resignation costing the organization $40-50K per nurse. Using the estimated costs of turnover and calculating the cost to adjust salaries in the targeted groups, he estimated the company could save six million dollars a year. For a non-profit that operates on a thin margin, this means millions of dollars going directly towards better patient care.
We’re always looking for levers. Among ten great ideas, “is pay a driver of retention” is one of them. Beyond adding new insights to market reviews, this analysis also created an educational opportunity to reinforce a key message: the pay grade is a powerful tool, so make sure initial offers are closer to the market midpoint.
During the pandemic, turnover went down significantly. But in 2021 Providence experienced higher than average turnover (as did many industries). The healthcare industry was hit extra hard by COVID-19. Providence has made incredible investments both to help caregivers receive paychecks even during the COVID shutdowns and now in attraction bonuses (>$220 million) to entice people to stay with the organization. The “Pay to Stay” project has been a successful piece to this complex puzzle. There are now fewer people in positions who aren’t below the midpoint, which means they’re much closer to having fixed this problem.
Because this study helped carve out the pay component of retention, Providence can use their data analysis to pinpoint other causes of attrition. For example, those paid above the midpoint have likely already been at the role a long time and have likely experienced different drivers and motivations. Maybe they want a promotion, or maybe their next step is retirement. For these people, money isn’t a great individual lever, but now Providence is better equipped to determine what is.
They also found value in very specific hotspots, not among global trends, which is a lesson for most organizations. Geography and type of job matters when it comes to things like sensitivity to pay. In Providence’s case, nurses and doctors were not the most likely roles to benefit from pay increases. Instead, light was shined on critical ancillary roles that were essential to high quality care across the hospital. Leaders had already heard about problems in those areas. Gary’s team provided quantitative insights to help leaders understand what actions would work.
The Pay-to-Stay concept has become a new tool in a toolbox, using survival analysis to test different drivers to see what other factors make a difference in retention. Gary and team are now diving in again with sign-on bonuses and retention bonuses. The success with this project has validated the approach of using data analysis to find root causes of costly problems and test hypotheses. Eventually he hopes to get the tools to the point where everyone in the organization feels comfortable using them to test hypotheses and find better solutions to running their organization. People analytics is a journey, and Gary says they are still far from reaching the end of the value it can provide to Providence and the patients they serve.
Results
$6M saved annually by upping pay
30% improved retention in key areas
< 1% of jobs analyzed were sensitive to pay