PeopleTech on the Edge | Ep. 02: Rethinking Talent Acquisition Architecture for the Agentic Era
Zack Johnson speaks with Michal Nowak, SVP of Engineering at SmartRecruiters, about the evolution of talent acquisition software and the transformative impact of AI on recruiting processes.
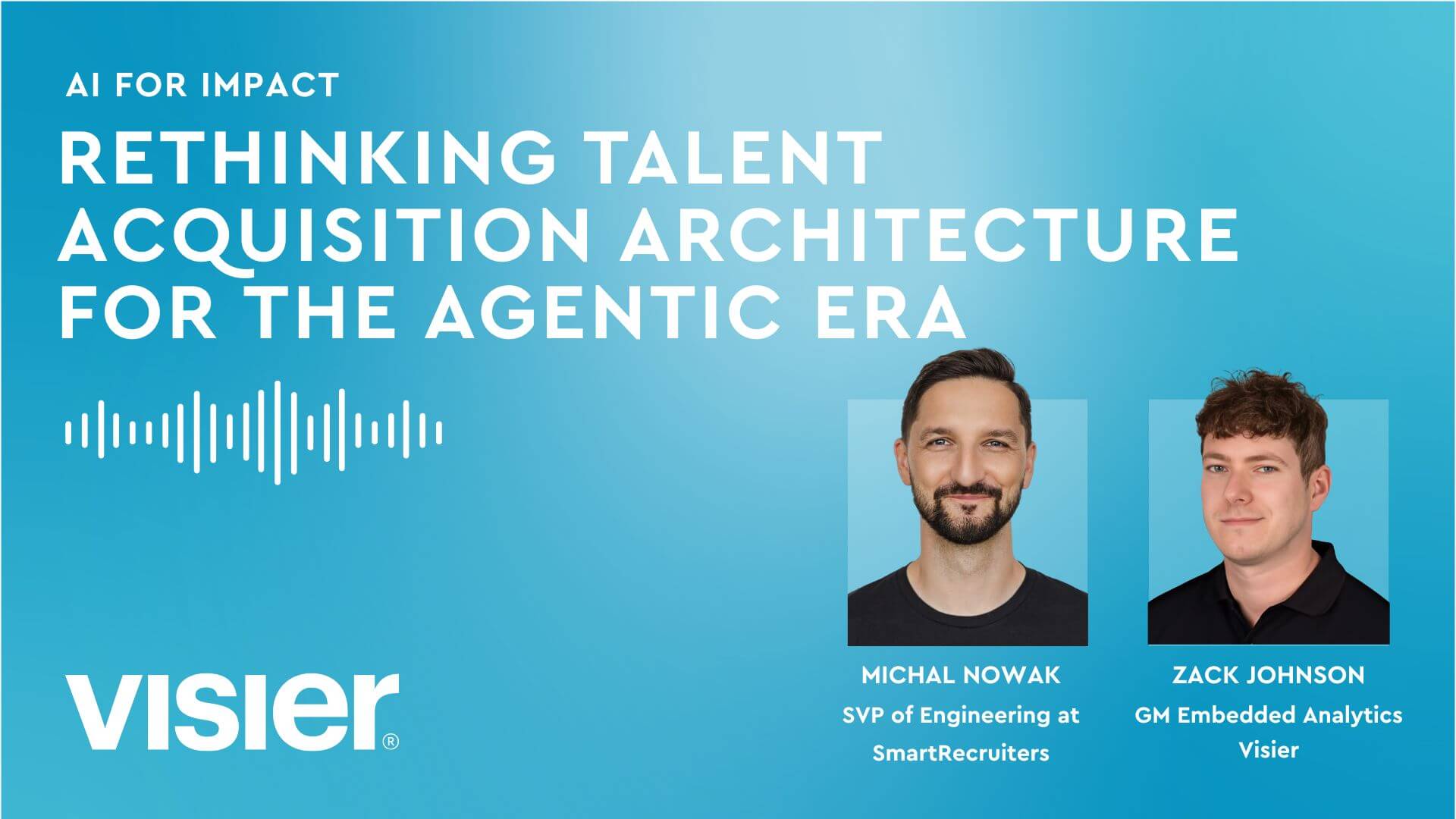
Rethinking talent acquisition architecture for the agentic era
Zack Johnson chats with SmartRecruiters' SVP of Engineering, Michal Nowak, dissecting the evolution of recruiting software and the AI revolution it's undergoing. They tackle everything from the impact of AI agents and ethical considerations to future-proofing tech stacks, all while Michal shares key insights on customer engagement and the enduring need for human connection in hiring, plus advice for tech newcomers.
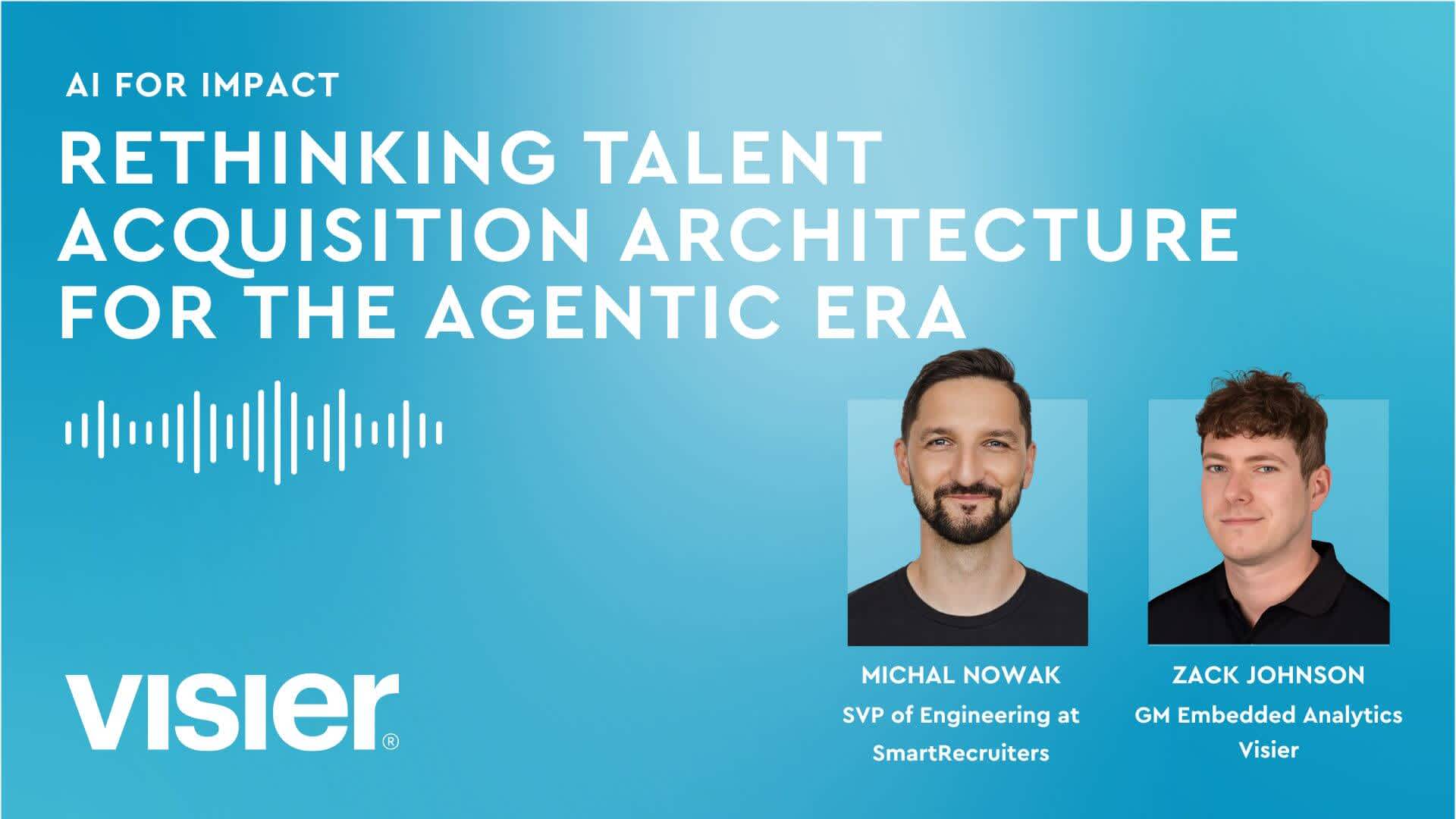
In this episode:
Host, Zack Johnson, General Manager, Embedded Analytics, Visier
Guest, Michal Nowak, SVP of Engineering, SmartRecruiters
Episode transcript:
Zack Johnson
Hello everyone, I'm Zack Johnson, GM of the Embedded Business at Visier, the leader in workforce AI and productivity. Welcome to another episode of PeopleTech on the Edge. PeopleTech on the Edge is a podcast where we come together and talk about how technology is constantly reshaping the world of work as we know it. Today, I'm joined with a very special guest, Michal Nowak from SmartRecruiters. Michal, how are you doing?
Michal Nowak
Thanks Zack, thanks for having me. Great to be here. I'm great.
Zack Johnson
You work in a really, really cool company, working on some really cool problems. Can you just tell us a little bit about who you are, what you do, and what brought you here?
Michal Nowak
100%. So hi again, my name is Michal Nowak. I am an SVP of engineering at Smart Recruiters. So we are building an AI recruiting platform. And in my capacity, I oversee engineering, which is like product development, infrastructure, security, and recently also customer support teams. And I joined company in 2012. like, yeah, I'm one of the dinosaurs here. I'm just starting my 14th year here. It's been like an eternity for a tech space. But yeah, I just love the experience and I stayed for so long. And yeah, I'm based in Krakow, Poland. And yeah, that's who I am.
Zack Johnson
That's an incredible journey to be with a company for that long. It speaks to your passion about the problem you solve. So what is it about hiring that captured your imagination?
Michal Nowak
So it changed a lot over time and the software in that space changed like dramatically over the years. Right. So like if you remember 2012, the first generation of that software and that we were replacing these was these tools were not really used by recruiters. These were just systems of record where people were filing in data, but no one was really actively using it as a work tech.
And then, so the second generation of these tools came and these were tools with really like consumer grade UI with building marketplaces, with open systems, with public API. And that was that second wave of talent acquisition software. And right now we're in front of the third wave, the third generation of talent acquisition software, which is like AI native, AI powered autonomous supporting recruiters. And this is like yet another shift we're seeing here. So like, yeah, throughout these 10, 14 years that I've been here, I saw a lot of these shifts and changes. And that's why it's been so exciting.
Zack Johnson (02:56)
That's amazing. And so if you think about like that first, you had like the three shifts, the first shift, was there something about the hiring process or like, you know, an experience you had interviewing or something like that, that like made you be like, ⁓ this problem needs to be solved.
Michal Nowak (03:13)
Yeah, I mean, there's just so much admin in recruiting, right? There's so much inefficiency in that process. The whole thing of like, you know, back then filling in a ton of forms and, you know, spending 30 minutes to apply and just not to mention crafting your CV and cover letter, but then also you enter the system that asks for like the same information that you have to retype it in the form.
And then, you know, the whole pain of scheduling and actually getting together with recruiters and, you know, setting this up and then, you know, applying and going through these multiple stages of the interview. there's, it was just hard. It was just hard to go through. And yeah, and a lot of these problems in the recruiting space are just meant to be solved through software, through good engineering and building with products, right? So that's why it's so exciting because a lot of these inefficiencies.
Michal Nowak (04:17)
Just great candidates to build software to help, and nowadays also to add AI to solve some of these issues.
Zack Johnson (04:36)
Totally. And so for a lot of people who familiar with smart recruiters, but for maybe those who are less familiar with smart recruiters, what kind of companies do you help improve their hiring processes?
Michal Nowak (04:30)
Yeah, I would say our sweet spot are large global multinational companies that are operating in multiple different areas. We've built a system that is just tailored towards supporting these large enterprises. It is just system that we build to flexible enough to adapt to various different hiring scenarios. So that was like, that's probably the one thing that I'm proud of.
But then there's others, right? Like I think we have a really solid UX and user experience. So this is really the system that our users are actually using and using it day to day. It is open. It has public API. We now launched our smart recruiters in Teams and Slack. So you can use the work tech to integrate with it. And yeah, and since last year we were like marching forward with a lot of AI use cases. We found actually a lot of problems in that space that can be elegantly solved using the AI and we're marching forward and shipping more of these.
Zack Johnson (05:41)
That's awesome. Thank you so much, so you describe these three phases of hiring software that you've lived through while building smart recruiters. This third one is it the craziest one you've seen yet? Like this AI transformation? Like it's gotta be just bananas right now building software in this environment.
Michal Nowak (06:00)
Yeah. I think everyone that I talk with, like all the recruiters that I talk with, our customers, they just see like a breakneck pace of like how things are changing around them. They just like, they feel like it's too fast. They are unable to just keep up with all the changes. And it's just like, you know, they cannot test all the tools, try them out. Like they're often like what do we need to do there? Which tools should we adopt? It is just changing so rapidly. So yeah, it is probably the craziest. Yeah, mean, crazy enough that we, last year, we just decided to halt everything. We decided to stop the roadmap and just rethink the whole thing.
Zack Johnson (06:45)
That's wild. So I want to ask you about what the experience was like halting the roadmap and like how did you even navigate that as an engineering organization? Cause that's, that's a big decision. But like, you think about the end user experience, right? Like we went from AI exists to everybody has a chat bot to like, we're going to have agents, but like what exactly is an agent? Like, how do you, how do you think about the role of agentic AI in the future of recruiting? Like, are you more excited about the workflows, the authoring, the decision making? Help me orient how you would think about some of those complex concepts.
Michal Nowak (07:25)
Yeah, yeah. So there are a couple of things that needs to be covered here. One, there is this notion of AI agents taking over large aspects of the apply process and the recruiting process. And I'm saying you have agents right now that can build your job description. You don't have to type it, obviously.
There is an agent, there's an AI tool that will create a good looking job description for you. There are other agents that take that job description, analyze it and build your CV that matches that job description, matches your skills and you got it. Now there is like a third group of agents that match these, you know, CVs to job descriptions. So it is that weird dynamic of like AI agents right now, working together and we're kind of on the sidelines, watching them how interact. And this is like a very bizarre thing to watch. And, you know, these users are beneficial to the candidates, for instance, so an AI agent can help you, you know, write a better CV, pinpoint the key things or leverage, like, you know, the skills, a relevant experience for you.
They can help you prep for the interview so you can have a mock interview with an agent right now or prep like, know, hey, figure out the questions that I'm most probably going to get asked. So these are like good things, but now you hear about, you know, fake applicants applying right now. I just read about like a Polish startup that almost hired a person that used like deep fake AI and was just really artificial CV, artificial LinkedIn profile, but also now artificial video interview and an avatar applying.
So for a companies like that are fully remote, it is a risk and it's really dangerous, right? To hire a person and then potentially like lose the company's secret information or lose money over this, right? So there are also like these dangers. So that's one part of the picture that, that, that dynamic over there from where I sit. And there's also a lot of human connection that right now we can build or build easier with AI tooling. So if you think about this process of scheduling, for instance, which is really tedious, really hard, and it needs to happen at scale, you need to just take into account multiple availabilities, it's a perfect job for an AI engine to do it for you, right? And this just helps get in front of the customer much faster, much more efficient, just to connect the human to human quicker, right?
There's also, you you can write more thoughtful messages. For instance, rejection messages. A large chunk of candidates will never hear back from recruiters from a company. They will never get any message at all. Right now with, you know, with these AI agents, you can not only respond to them, but respond to them in a thoughtful manner taking into account the interview feedback. So this actually gives some value to these candidates, right? And then, you know, I talked about like CV creation, but also like these agents can surface the relevant skills, the relevant opportunities. Like if you have 1000 candidates and that huge list of people that applied for your job, there will be like, you know, these agents will help you surface relevant candidates, right? So, you know, they're providing a more equal chance of getting employed. And that's also one of reasons like this, this matching thing that this needs AI systems needs to be built in a very like responsible, thoughtful manner. But yeah, this is the, this is the landscape.
Zack Johnson (11:21)
Super, super cool. And thank you for going deep on each of those. So there's a couple, there are like a few big themes that emerged from that on like the value that AI can provide and also some big themes on like the risks and challenges that you're in real time to having with your customers. So let's see if I got some of those. So on the value side.
The same way you said there's all this admin back in the early phases, it's like there's still that admin. So whether it's scheduling or parsing through things, et cetera, AI is going to be able to, in a lot of ways, do that better than a human can. More quickly, more effectively, more efficiently, which is going to maximize time spent on things that provide value. That seemed like one. The second one was there's a big theme of experience. So like, I am a candidate.
Am I being talked to like I'm a human? And it's kind of ironic that like AI is the unlock for that experience. like, you know, I think about like two years ago, three years ago, like the ghosting of candidates that became like a, like a TikTok meme and like, you know, how you, how you're handling rejection and how you're doing prep and all those things. so in a lot of ways you can have like a hiring coach, right? The only one you didn't bring up is like hiring decision-making, which I do think is a opportunity and also challenge, right? Cause you have to watch out for, for, some of the most like targeted AI use cases under scrutiny or like choosing a particular candidate. you have AI make that choice that breaks the law in some places. like big opportunity, big challenge, but it was also a question where like, if you're looking at 200 candidates for one job, like who's the person who's going to be most likely to be awesome at that job?
Like there's a lot of interesting data in the outside world on the inside world, et cetera, to be connected. But it's a wild time. Like all that opportunity, all that risk. And like you said, the customer's kind of overwhelmed, right? It's like every day there's a new innovation.
Michal Nowak (13:18)
100%. Yeah. So that, you know, that automated decision-making, right? Like this is something that, well, we basically, I feel like we cannot do that for recruiters, right? So there needs to be a human oversight and this is the big challenge. So if you think about it, these AI systems right now, the AI system that we build, they will surface skills. They will surface some information from a CV and just highlight them. It will mark some of these skills are matching in green. Some of them might be yellow, right? Or there might be a, you know, they might identify a perfect matching candidates for your job, right? And even if these systems will not decide on behalf of recruiters, they will influence their thinking, right? They will prompt them for, you know, some choices to be made.
And then if you think about it, this just happens on the large scale, right? Potentially to millions and millions of candidates. So we feel like tremendous weight on our shoulders to make sure these systems are ethical and responsible. And it's just a huge task and a big thing that you need to like design in into those systems, right? So there's just a lot of thought and a lot of effort to make sure like for these use cases, we're building the safest of those ethical systems that we can build. Yeah, because in AI and recruiting, you think what is the risky use of AI and people will tell you the healthcare, credit scoring and recruiting, right? These are some of the most risky applications of AI.
Zack Johnson (15:06)
And I love that you summarize that with like the healthcare credit score and recruiting, because you can make a structural decision that impacts somebody's life and livelihood. And it's like, you can't look and there's no accountability, right? it takes a lot of stewardship to build software that's thoughtful, mindful, and compliant, especially as like the regulatory landscapes that complete moving target and also it's gonna be different in different regions. So if your customers are large multinationals, how do you set something up that's gonna be ahead of where things are gonna go, still give you that technology edge, but also be something that you can trust you can roll out. I think that's one of the challenges of building at scale B2B tech, particularly enterprise tech right now, is how do you do all those things really well? And it's hard, it's really, really hard. So I have a lot of respect for what you're building and trying to do.
What's funny is the risk really is a two-way street though. There's the risk that a candidate gets passed over or put through something that's unfair. There's also the risk for company, like you said, you've got deepfakes, have candidates who are misrepresenting their experience but doing so extremely credibly. But actually one of ones that's kind of funny, I've even seen this in interviews, if you have any sort of pre-work for an interview or you do project-based stuff, the project sometimes will be 100 % done by AI.
in some ways is good. It shows the person's using AI creatively to do the job, but in some ways the whole point of it is to reflect the understanding. And so there's this catch-22 where it's like, and I think this creates some of the real, there's talking about the technology and what the technology does, but if you also look at talent management at a macro level in the world, you need to like...
Like when I started my career, I was doing super basic data entry, like on the side for extra money, which actually helped really inform my understanding of how data models worked. Cause if you're literally messing around with like binary, if you're fact checking binary data sets for like network, always doing it with network analysis, I really understood where the network came from. Challenges is if from day one, you can use AI to do super advanced problems. Do you learn some of the like foundational skills that are required to then build upon it? Otherwise you just build on air. And so I do think there's like this weird agentic loop in hiring a little bit, right? Where it's like an AI takes the JD, writes a resume, writes a cover letter, you interview, the interview is evaluated the same way. And so like, it's, I don't know, it makes me, I think about it little philosophically, which is like, where's it all gonna settle? I don't know if it'll ever settle.
Michal Nowak (17:56)
Yeah, it's a tough question, right? like, but they're like, so just for the safety of the AI system, there are things you can do here, right? So it's not like we're helpless. It is, I think, first of all, you need to design with customers for the use case like this, that is potentially risky. You need to always design with the customers and make sure like you're building something they're comfortable using, right? And this is something that, you know, they're, they want to use, they know how this system behaves and they know what to expect. And we just are blessed with customers that are super passionate about this tech.
They give us feedback all the time and just spend countless hours with us, with the design partner program, and then just basically really build that software with us. I think like also you need to make sure these systems are explainable. So like it cannot be like a magical box then that just spits out outputs, you need to make sure this system like that has explainability built in. And you can just trace back, you know, why these skills have been highlighted, why the score has been like, looks like this, right? You need to be able to comprehend that. And then finally, you need to test it, right? You need to test the agent, right? If you treat your AI agent like an intern helping you.
You wouldn't hire an intern without checking anything, right? You usually run some for this interview process, right? And then the same thing is happening right now with an agents. And we are fortunate to partner with super smart companies that are actually testing agent trustworthiness. And it's such an interesting topic because it's, it's, there's so many aspects to it, right? There's like the notion of bias, right? And then racial, gender, disability, location, institutional, there are like all these different forms of bias that you need to be aware of. Or like stereotype, if the agent is like, you know, engaged in a stereotypic behavior or toxicity, right? If the agent uses profanity or not, like robustness, like, you you just ask the agent for the same thing and it just more or less responds in a similar manner, which is super tough for like in gen AI that are like undeterministic.
So yeah, but you can test for all of these and you can try to just validate this and verify and you need to put a lot of effort into this, right? So these are some of the techniques, some of the considerations that we're certainly having before shipping that software.
Zack Johnson (20:35)
Totally. I mean, we're seeing some of the same things just when it comes to like using data, right? With AI, because you can throw whatever data you want and AI will generally come to its own conclusion. So you have to make sure that the observability is super high. You also have to make sure that like it's, there has to be like a contextual data model. Otherwise, like you're making up the numbers you're using to do the math in the first place, which becomes a huge problem. And so it's similar themes applied in different ways, right? But the, you know, so you mentioned earlier, when the big AI my god moment came you paused the roadmap like and I believe you told me you paused the roadmap it was for like three months of releases just like pause so how are you leading through this moment as a technologist as a the exact like talk me through like that moment and like how you're thinking about the stuff now
Michal Nowak (21:26)
Yeah, yeah, that was great. We looked at a road map and like the pace of the LTS advancement just got to a point where we look at the road map and thought to ourselves, look, are these features going to be even relevant like a year from now? Will anyone use it? And we decided that like we need to rethink all of that and just get back to the drawing board. We told our customers that there will be no releases for three months. We're just pausing everything. And we took 30 % of the team and we sent them for an aggressive discovery work. So we picked up a bunch of engineers, product managers, designers, and they were just swarming our customers and doing customer interview after interview.
We did dozens and dozens and dozens of these interviews just to really uncover these problems like second time. Let's just do a second look and add everything that we wanted to build and let's just look at it through the lens of like this emerging technology. Right, so we had 30 % of the team doing this and really the remaining 70 % the engineers, they were sharpening the axe. So we were rewriting both our front-end and back-end tech stack and we were trying to optimize it so that it's just better suited for AI assistant coding.
So we decided just to, need to standardize the tech stack even more. We were never like having like too many technologies, but we went on standardized on standardized the backend tech stack around Java and backend frameworks. And with the front end, did the TypeScript and we rolled out our design system and made sure really that the tech stack that we're ending up with will be like easy to integrate with these AI Assistant developer tools. So we try to just standardize around the most popular framework where there is the most training data. And that's what we shifted the whole tech stack. Yeah, so that was the... And then we spent a quarter doing this and afterwards the Winston was born. So that quarter we designed the Winston, the AI Assistant.
Zack Johnson (23:53)
That's amazing. Thank you so much for sharing. like, and I hope you don't mind if I ask a couple questions about that journey. Cause like the, I think there's a lot of people who are in various stages of journey themselves, right? So like a whole bunch of companies basically took a tiger team, spun them out and said, start from scratch. Some people said, let's go in and just modify our current platform by injecting AI in, whether it's like a co-pilot integration or what have you at various levels of, architectural significance. What's really cool is when you talk about re-platforming front-end and back-end at a time when the technology is changing fast that nobody has the expertise yet. Like the best practices for AI ready are like in flux at the same time. Like how did you build that expertise as a team? Like how did you make those architectural decisions? Like I'm just curious if you learned anything there that might be helpful for other people doing the same thing.
Michal Nowak (24:48)
Yeah. So that productivity work we did, it's just like, you know, we try to future prove us and just, you know, optimize around the tech stack that has a potential to be like easier to work with AI. And then when it comes to like the team and skillset, yeah, I mean, we had some skillset in-house because we were building AI systems before like starting 2017, but then we needed to just scale it and just really like grow that skills and the amount of that skills within the team. think like there are a couple of important things here. like one is really ability to run the discovery and I mean product discovery and identify good use cases for AI, right? So like that is something that all of the product teams should adopt right now and try to figure out the good use cases, right? What might be?
The high potential or like good use cases for the use of AI. So things like, know, and in general, things where there's a lot of repeated activity, right, that happening all the time, where there's a need to analyze a lot of data. And when your problem is not, when imperfection is not a problem because these tools are so like, you know, non-deterministic.
You can ask an AI system like six times about the same thing and you you got five answers and the sixth one is a different. So like if your problem is like, know, immune to that imperfection, okay, there might be a good use case for using that technology, right? So we try to just make sure that every product team can understand how to identify these problems and run a discovery, right? And that's one thing we did. The other thing is, we did a lot of partnerships with folks like AWS.
They have an amazing team running prototyping. And we just spent a lot of time with them prototyping together using. So we're standardizing over AWS. And these folks really helped us to kickstart, bootstrap some of these efforts. And that was really helpful. Also, there's a lot of great tools out there. for instance, for like managing internally for managing conversation flow, use Rasa as a framework and it also like helped us jumpstart and just, you help us build something. like, yeah, using the tools and partnerships to inject that knowledge was the second big thing. And third really is around the engineering productivity and basically making sure our engineers can leverage these tools to their benefit to improve their life. And that's like, you know, a big area of like training and onboarding and cross training with the team. So yeah, that will be these three elements.
Zack Johnson (27:59)
Thank you so much. think the journey is pretty wild because a lot of our companies experience the same way. We're like, everybody had that first magical moment. And some people were earlier to LLMs, in particular, than others. And there's also people who have been building machine learning models for a long time now. So there's all sorts of different applications of AI. that software development has been very deterministic for a long time. You set something up.
You run QA, you see what breaks and you fix it and you run it again. And then you see if it's actually fixed. Running something that's non-deterministic, it's really crazy. You the irony is it's a lot like people management, right? Like humans are non-deterministic. you know, you don't, you don't rate people's performance on a hundred percent outcome most of the time. It's are they right most of the time? Like, do they make good decisions when they encounter different problems? And so it's been really interesting seeing the discipline of software development.
Go from something that's like extremely hardline determinist engineer to non-determinist like almost psychologist, you know? Where it's like, I'm just trying to cajole the system into being consistent enough for me to then actually set my workflow together. And so it's an interesting skill time. I'm curious. So like you get to spend, you had a big focus on listening to customers and listening to their use cases and working with customers to make sure it clicks. What are you hearing from customers or seeing at customers that get the deterministic versus non-deterministic thing? Are some customers just getting it right early, able to adopt the technology and experiment and others are stuck? How are you seeing that dynamic play out when you actually roll out features?
Michal Nowak (29:45)
Yeah, there's a whole variety of responses, There are customers that are like really open to test a lot of different things and they're trying it out and just, you know, accepting some of the risk of like using early products in their alpha version and just really trying a lot of different tools. And, you know, and having that, you know, that, that experiment or mine, there's a lot of customers that are naturally, you know, more cautious and you know, they're like, and for a good reason, right? They're businesses like that, that are working on like nuclear power plants and like, know, hiring people there or like, you know, government agencies or like, you know, labs. So, and they're like more cautious and they want to have like, you know, a better, more options for like rolling out the software for a small sample of users, trying them out in a limited scope.
They're also like really digging hard into how these systems are being built. So most of the companies right now also built their own AI governance or AI working groups. And these teams are building like, you know, the procurement processes. And this is like great because we are we have a conversations, are often offering us tests or they're like, you know, sharing how they think about evaluating the software. So this helps us also like, you know, respond to that and build better, better systems.
So yeah, that's that. And so there's a wide spectrum of how people are adopting and using these systems. The one thing that is just like commonly brought up is like adopting the conversational way of working or chat to do the recruiting. This is not something common. This is not something that we're used to, right? It's a different way of interacting with the talent acquisition software. So yeah, that is something that we're also trying to figure out. And there's just a lot of aspects here, like when the chat will pop up, how it presents itself and when, what are these points in the life cycle of the product that mandate, hey, I'm here to help or hey, I noticed something interesting. Let's have a conversation, right? And then the other thing is not everything should be like a conversation. We're also like spending a lot of time figuring out how to render UI within the chat and do the adaptive UI because there's a lot of use cases where you should not just type all the comments, right? So if the chat asks you, where do you live?
You should render a map and allow people to click on it, right? Or if you want to upload a file, of course, like there will be file upload or if you fill in the form, then you should be able to fill out a form and just dynamically build it. So that's, you know, that's another thing that helps you adopt the software. And the third thing is like we're thinking about is really like, you know, getting the software into work tech, right? Embedding our agent within Teams or Slack where you've often like, you know, spend most of your day and you chat in these tools, right? So this is just like more natural environment for these, for these tools.
Zack Johnson (33:02)
Yeah, absolutely. You know, it's funny because like, I think there's going to be, there's recurring themes I'm seeing appear, talking to people, building technology and implementing technology across a wide variety of organizations. One of them, which you just brought up to medium, which is, is everything a chat? Is everything a voice input? Like, are people using things to click maps? Like it's kind of anyone's guess. And so you have to be prepared for being wrong, think, like as a builder, like, you know what I mean?
Cause like, like, you know, you're, and this goes back to the work tech thing. Like you're not going to supplant teams as the dominant screen or medium like, how do you integrate? What happens if teams like totally shifts paradigms? Right? Like, and so part of that's like how flexible you can be. I think the other one too, which is a really fascinating question I've been spending a lot of time thinking about is like, what are the units of work? So like, you know, as someone who like manages people and has a decent size org, the meeting has become a really important unit of work. Like you have a half an hour meeting, you have an hour meeting, got rid of you of a two hour meeting. What can you get done? You know, you get two hours of quiet work. What does it look like?
It's weird picturing work being like stream of conscious and slack. Like, yes, there's all this back and forth, but like slack's horrible for actually managing workflow. Right? And so like, how do we take these tools that are so conversational and lacking structure and then create new forms of structure to be able to manage them or are the tools going to actually manage us? Right? And so like, I think there's some really cool, I mean, manage us not in like the like, you know, the machines take over way, but just like, does a good day of work look like? Like a good day of school is established, but a good day of work changes based on your role, based on your seniority, based on the type of industry you're in, based on a whole bunch of different things. And so it's really cool that you get to work in a business that cuts across all these different types of work globally to do the same process each time that the process can differ. It's wild what you're getting to work on right now. I'm excited to know you, man. It's really cool.
Michal Nowak (35:15)
Yeah, it is super interesting. It's been super interesting. And if you would talk with our product designers, I think for them, that's even bigger shift than for engineers. How to design a conversation that is well structured, that can be followed, that is less ad or prong, that will get you where you want. And you know, where do you wish we have a conversation versus render a UI? This is like what they're thinking about right now. And that shift is just massive. We were shocked like, you know, how different it is to design it.
So yeah, this, this is big, right? And it, you know, a lot of the UI will be gone. If you think about scheduling, rendering a calendar, like, you know, adding people in comparing who's available when it's just getting super complex, super fast. And if you think about just typing, hey, I'm out of office this week, move all my meetings for the next one. It's just so much easier, right? It's easy, like, I don't know, 200 hours just saved on doing all of that, you know, manual clicking. So yeah, I think there are use cases that are just excellent fitted for that conversation.
Zack Johnson (36:36)
I don't think it's been a better time to build technology because it's so exciting and you can become an expert so fast because everybody else, no one has like 20 years of expertise on this. Like it's totally new. And so you can go from zero to like really being quite expert and influential really fast. And like, you know, it's funny because like, if you think about the levers of business, you basically have like the levers for building a business or building an organization from inside, right? You have people, process, technology and like, people processing technology are going to fundamentally change because of AI.
And so you've such a moving target and like, like kids for lack of a better word, we're going to make up entirely new organization models. We're going to make up entirely new ways of organizing and operating. And some of them will be self originating. And it's just, it reminds me of like, we've seen a few technology booms. Like I used to work in like music marketing and like entertainment stuff. And when social media came out, no one was an expert on social media except people who use social media, which are mostly young people. And so you actually have this really cool boom where like it was an amazing way to start a career and like create a footprint because you were the expert and adults would listen to you and it was awesome. And I think AI is similar. And so the last question I just want to ask you was, do you have any advice for people who are either getting into tech or rethinking what they're doing in tech with regards to all this?
Michal Nowak (38:02)
Yeah, yeah. It is interesting, right? It's AI, it's been as of today, as of March, 2025, AI serves mostly the senior people and really like experienced users because of that 70 % rule that, you know, Adios money from Google, he coined it. And it's basically says, there's AI will take you 70 % there, but the last 30 % actually will you just spend most of your time polishing your product, making sure it's production ready, it's performant, it's secure, it's usable. The quality is there, right?
And this just takes a ton of time. So as of today, that 30 % still exists. And if you look at how senior people work with AI right now, they just, you know, there is a generated code and but they constantly rework it then constantly improve it they just talk with the machine and use it as a tool but then you know never blindly trust the outcomes I think but it is for the people that are just getting into tech I would say really focus on fundamentals on like really deep understanding of what's underneath the frameworks and languages. The differences between languages and frameworks are now becoming really so much easier to grasp. The differences in using different technologies and languages, I mean, these A assistant tools, they will get you there. They will explain a lot of these concepts or help you migrate between languages and frameworks or generate a lot of code for you.
But you need to understand the fundamentals and the concepts and the foundation of it, right? So you need to, as of today, you need to understand what's being worked on. And I think the other thing that is just becoming extremely important right now is the ability to work across functions and for engineers to be able to work effectively, not only across functions like product and design.
But also together with customers and understanding the business and domain and communicate clearly. There's so much more emphasis on using like, you know, the English language to interact with machine, you know, writing thoughtful prompts or like, you know, pointing out to the machine what needs to be generated that, you know, English is becoming the hottest programming language of 2025. And, you know, the clarity of communication, the structure is becoming extremely important. this is something like, know, writing well is a skill that is more and more important right now, I think. So yeah, these are like these two advice I would give. Yeah. It's a different world for these people, for sure.
Zack Johnson (41:0)
It's a different world. I think one that was coming out through our conversation too is like, you know, so much of the AI boom around software was how we take existing process and like AI-ify it. But really quickly it's turned into what would this look like if the original process didn't exist?
What would this look like if the original assumptions weren't there? Which is crazy because it's pure invention. And that takes creativity in beginner's mind. And so the combination is tricky. How do you, like you said, have something that gets you 70 % of the way there that you were growing up using, still be able to learn the 30%, right? Without necessarily doing all the legwork, but also be in a place where there's infinite creative options. It's really quite tricky, but it's exciting, right? And I find with great challenging chaos comes opportunity, but it's tough.
Michal Nowak (41:55)
It is, but also like learning right now, the barrier to learn is just so much lower. with these new tools, right? That do a deep research for you. You're starting a project, you're thinking about what would it be the best architecture. And you can have that tool basically go through and run a deep search and just in a fraction of time, tell you, hey, most probably this is what you should do. And you know, it's a tool, you use it as an asset, a tool to help you out, but it's such a boost to be able to quickly understand what's out there and how to structure your thinking. It's just amazing.
Zack Johnson (42:35)
Just amazing. Well, thank you, my friend. I really, really appreciate you coming and joining me on PeopleTech on the Edge. It's a great conversation. We covered so much ground from like the phases you've seen the recruiting world going through to like how you and your team dealt with like the AI boom and have thought about it with your own roadmap to like advice for people who are building product. And of course, how AI and modern technology is transforming like the recruiting and job applicant process.
So thank you so much for taking time. Thank you for taking time late at night. And I'm really glad we got to sit down and talk. This was a great conversation.
Michal Nowak (43:10)
Zack, thank you so much. It was great speaking to you. Thanks for having me on the podcast.