
DATA ARCHITECTURE
Data Mesh vs. Data Fabric: What’s Best for Your HR Data Strategy?
The analytics tech ecosystem is always growing and evolving. This series guides technical stakeholders so they can better understand how easy it is to uplevel their data stack with Visier.
Start free trialTable of contents
Problems with centralized data ownership Data mesh vs. data fabricVisier fits into your data mesh or data fabricData is a powerful resource that can give you a competitive edge in the market. And that’s why we should manage it well. With data streaming in from diverse, unstructured sources, it's become increasingly challenging to securely consolidate, sort, filter, and process it.
Traditional monolithic centralized architecture is cumbersome, expensive, and inflexible. So today, technical teams focus more on the data mesh or data fabric concept. This modern approach to data management tailors technology to each business area, enhancing data sharing and usage for improved decision-making.
With the rise of generative AI, data management becomes even more critical. In this post, we'll discuss why centralized data management is obsolete, compare data mesh and data fabric, and outline how Visier supports both strategies.
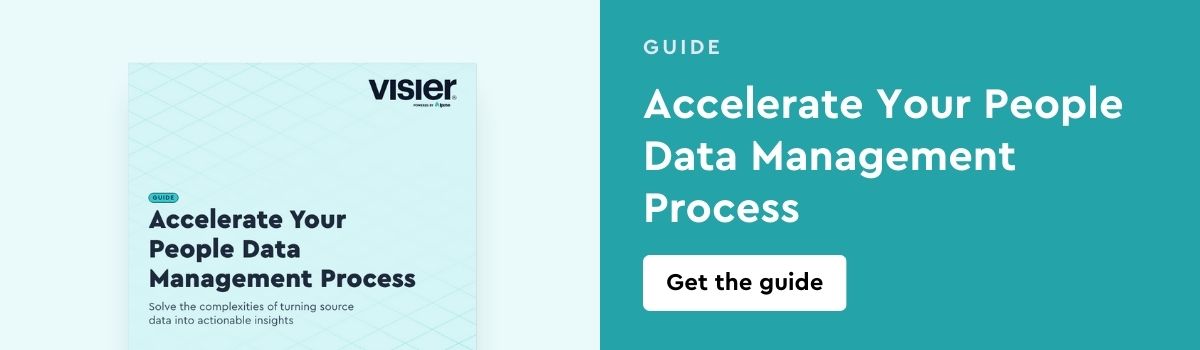
Problems with centralized data ownership
Initially, centralized data ownership offered advantages like faster data analysis, but all this placed a heavy burden on one team. As data volumes grow, maintaining agility becomes costly and requests take longer to handle.
Within this ecosystem, traditional centralized systems often fail to scale effectively because:
Siloed teams impact data accuracy. Centralized data scientists and engineers lack business and domain knowledge, leading to inaccurate insights.
Slow responsiveness to change. Data engineers implement pipelines with limited domain knowledge, requiring heavy modifications to the entire pipeline when any change is needed.
Centralized-only approaches also lack domain expertise. For instance, while a data engineer may excel in various data types, they may not grasp the nuances of specific domains like HR—especially when connecting data points along an employee's journey.
The data mesh approach allows IT teams to govern the centralized platform while empowering domain areas like HR to control their data. This benefits HR, ensuring data ownership and tech selection, but also means that IT can receive expertly crafted data for the centralized system.
Despite investments in next-gen data lake or cloud data warehouse architectures, many organizations struggle with scale, speed, and organizational intricacies due to reliance on centralized data models. This delays time to insight and continues to complicate the data management architecture.
Transitioning to a data mesh or data fabric marks the next step in the evolution of large-scale data management. These methodologies aim to expedite the creation of data products and enhance data accessibility for businesses.

Data mesh vs. data fabric
What is a data mesh?
A data mesh is a modern data architecture principle founded by Zhamak Dehghani in 2019 that treats data as a product. It views datasets as distributed products oriented around domains, such as HR or Finance.
In this approach, IT retains centralized data ownership while facilitating data movement across domains and into the central data repository. Each domain independently manages its datasets, encompassing both raw data and data products, thereby fostering greater ownership and accountability.
This is how the founder defines a data mesh:
“A decentralized sociotechnical approach to sharing, accessing, and managing analytical data in complex and large-scale environments—within or across organizations.”
Zhamak Dehghani, Principal Technology Consultant, Thoughtworks and architect of the term “data mesh”
A data mesh enhances efficiency by improving data access, security, and scalability. This includes a data catalog storing metadata like table names, columns, and user-defined tags.
Here's a quick overview of what it is and what it’s not:
A data mesh is | A data mesh is not |
---|---|
A set of guiding principles | A specific vendor technology or product |
A domain-oriented, decentralized approach to data ownership and architecture | A data warehouse, data lake, or lakehouse These are complementary and may be a part of a larger data mesh that spans multiple lakes, ponds, and operational systems of record |
A method for treating data as a product | A one-off consulting project |
A self-serve data infrastructure as a service | A data catalog or graph |
An implementation of federated governance for effective management across domains | A data integration solution (A data mesh doesn’t bring data together in the same way a data store would) |
A self-service analytics product |
A well-executed data mesh offers several advantages:
Accelerates time-to-market for data analytics
Democratizes data to speed up decision-making
Enhances data IQ through increased engagement and learning, and
Provides transparency by allowing domains to choose their ideal tech stack
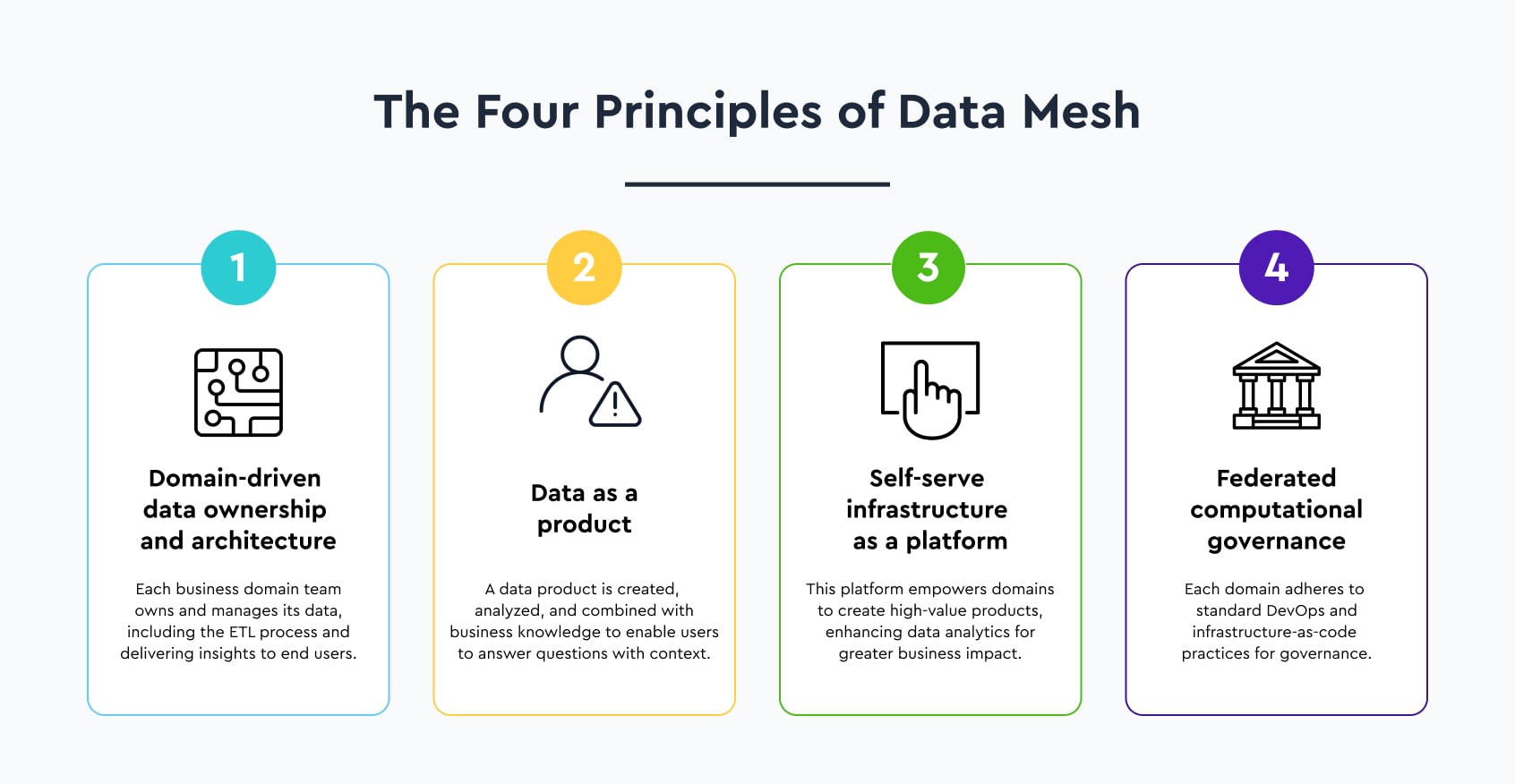
What is a data fabric?
According to IBM, a data fabric is “an architecture that facilitates the end-to-end integration of various data pipelines and cloud environments through the use of intelligent and automated systems.” It connects all data sources and reunites data matching, observability, master data management, data quality, real-time data integration, and more—without requiring a complete overhaul of your current tech stack.
Gartner advocates for deploying an integrated layer to accelerate generative artificial intelligence (AI) adoption, offering a single API access point and governance. This unified infrastructure layer allows secure use of generative AI across all data sources, limiting AI to trusted data only.
The following are important considerations for deploying a data fabric:
Collect and analyze all types of metadata (technical, business, operational, social) to create a well-connected pool.
Transform passive metadata to active by:
Continuously analyzing metadata to extract key metrics and building a graph model.
Visualizing metadata graphically to highlight business-relevant connections.
Enable the creation and management of knowledge graphs to add semantic context, enhancing AI/ML analytics.
Ensure a robust integration backbone compatible with various data delivery styles.
Here’s a quick comparison to help you understand the differences between these data architecture methodologies:
Data mesh is a distributed business and development architecture | Data fabric is a distributed data architecture |
---|---|
Shifts data management and development to domains | Offers a single view across all your data |
Eliminates the sole reliance on centralized data warehouses, data lakes, or data lakehouses for domain-specific needs | Supplements a data warehouse, data lake, or lakehouse |
Provides greater flexibility in centralized data architecture |
The adoption of data mesh and data fabric architectures is growing
A Gartner survey found that 38% of CDAOs plan to overhaul their data and analytics architecture in the next 12-18 months.
Currently, only 1.5% of the global IT market uses a data mesh, and just 18% of organizations are mature enough to adopt it.
Despite its early market stage, many IT departments see a data mesh or data fabric as viable solutions. IDC predicts that by 2025, 80% of organizations will have adopted a data fabric.
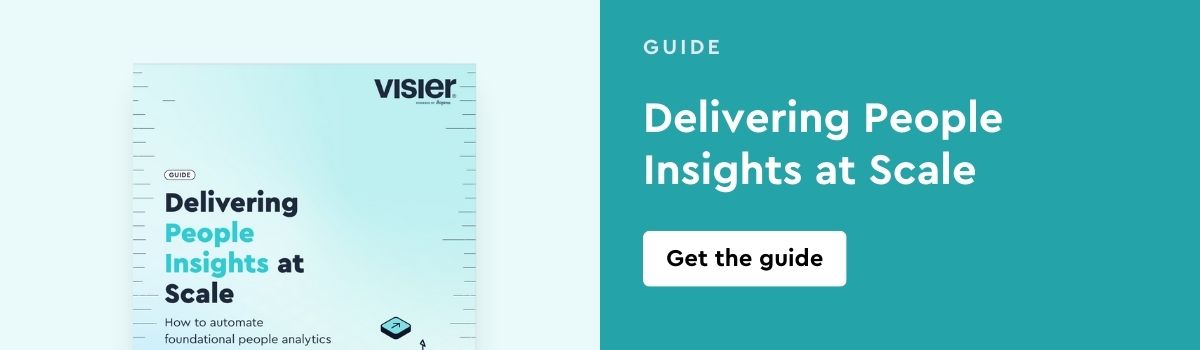
Visier fits into your data mesh or data fabric
Organizations have multiple data sources that need integration for analytics. This is particularly challenging with HR data due to its time-based nature, sensitivity, and evolving workforce dynamics. Traditional data management approaches remain inadequate, and most enterprises lack the expertise to handle complex people data.
Visier is best positioned to be your data mesh or be part of your data fabric for people data within the HR domain, offering industry-leading practices for success. It acts as a semantic layer and people data mart across your HR data stack, offering a single source of truth. It serves as the mesh or fabric component for HR, providing scalable insights even beyond HR. With Visier, organizations gain a trusted data foundation for HR data and simplified access to any source. Admins save time with built-in prototyping tools, reusable examples, tutorials, and workflows for scalable people analytics.
Adopting, building, and maintaining a data mesh or fabric requires significant effort. Visier People empowers self-service HR analytics, allowing operational data to be layered on top for workforce insights. This gives domain teams full control over their data and analytic model, and rapidly transforms messy data into actionable insights.
Domain expertise is crucial for success, and Visier provides the HR expertise needed. Trusted by over 50,000 customers in 75 countries, including BASF, Panasonic, and Ford, Visier helps you make better workforce decisions.
Want to learn more? Try Visier with our 30-day trial and see how we can support your HR domain.